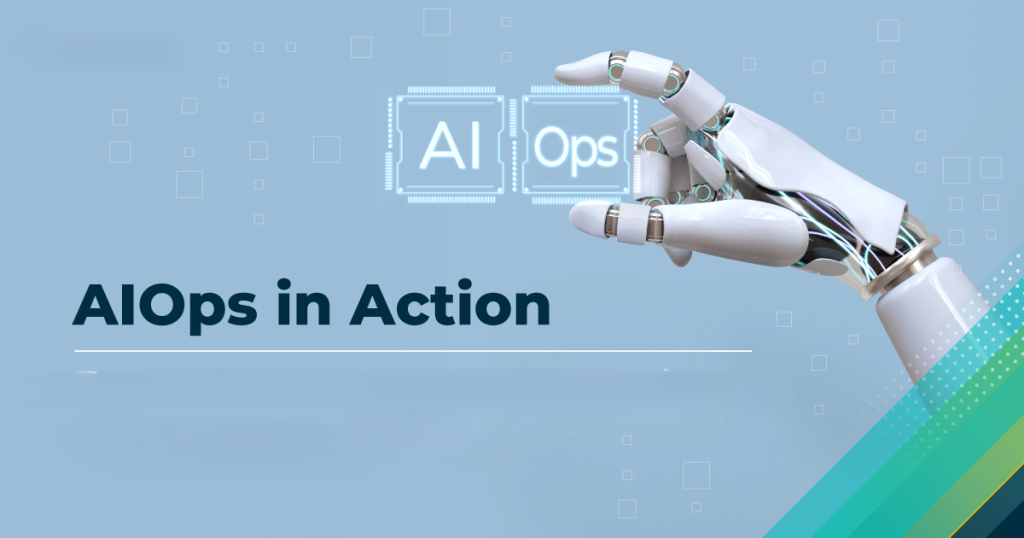
Introduction: Moving from Reactive to Predictive IT Operations
Outages remain one of the biggest threats to modern digital businesses. Whether itโs an application failure, infrastructure misconfiguration, or cascading service degradation, unplanned outages disrupt revenue, erode customer trust, and hinder digital transformation efforts.
For years, IT teams have relied on reactive monitoring tools to detect and address outages after they happen. However, in todayโs complex IT environments โ spanning multi-cloud platforms, microservices architectures, and hybrid deployments โ traditional monitoring approaches simply cannot keep pace with the speed, scale, and complexity of modern IT operations.
This is where Predictive AiOps (Artificial Intelligence for IT Operations) is transforming outage prevention. By combining real-time observability, machine learning-powered forecasting, and automated response capabilities, Predictive AiOps equips IT teams to spot, predict, and prevent outages before they impact users.
Why Predictive AiOps is Crucial for Modern IT Operations
- Detects subtle risks before they escalate into full outages.
- Provides real-time analysis of cross-domain dependencies.
- Uses historical data to predict future performance degradations.
- Triggers automated corrective actions to avoid disruptions.
- Aligns IT reliability with business continuity goals.
Key Features of Predictive AiOps in Outage Prevention
Predictive AiOps is not just faster monitoring; itโs an advanced system that continuously learns, anticipates, and proactively manages IT health. Its capabilities span data ingestion, machine learning analysis, predictive modeling, and intelligent automation.
Major Features of Predictive AiOps
- Cross-Environment Data Aggregation
- Collects data from applications, infrastructure, networks, containers, and cloud platforms.
- Normalizes data into a central observability layer for real-time correlation.
- Ingests structured data (metrics) and unstructured data (logs, events) for holistic analysis.
- Dynamic Baseline and Pattern Learning
- Establishes performance baselines for every system, service, and environment.
- Learns how systems behave under normal, peak, and failure conditions.
- Continuously refines baselines to account for seasonal traffic, new deployments, and business trends.
- Anomaly Detection and Multi-Layer Correlation
- Identifies subtle anomalies in performance, configuration drift, and error patterns.
- Correlates anomalies across dependent services and infrastructure layers to detect systemic risks.
- Predicts potential cascading failures by mapping service interdependencies.
- Predictive Risk Forecasting and Early Warning Alerts
- Predicts likely outages based on historical incident patterns and evolving anomalies.
- Provides risk scores to prioritize high-impact services and business-critical systems.
- Issues early alerts with probable root causes and recommended preventive actions.
- Automated Remediation and Self-Healing Workflows
- Triggers automated prevention workflows for predictable failure scenarios.
- Performs preemptive actions like scaling, service restarts, or configuration adjustments.
- Learns from successful and failed interventions to refine future prevention strategies.
The Role of Historical Data in Predictive AiOps
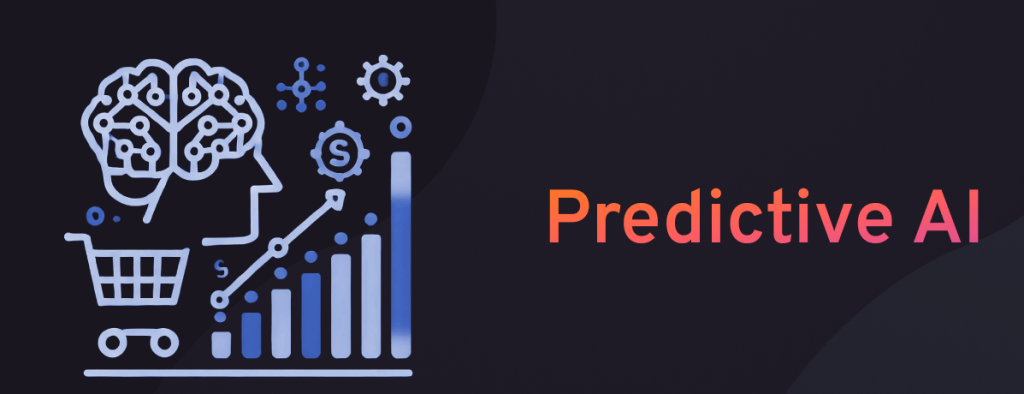
One of the key enablers of predictive outage prevention is AiOpsโ ability to learn from past events. Historical performance data, incident timelines, root causes, and remediation outcomes train AiOps algorithms to predict future risks more accurately.
How Historical Data Powers Outage Prediction
- Identifies recurring pre-outage patterns across systems and environments.
- Links common triggers โ configuration drift, resource saturation, deployment issues โ to past outages.
- Maps dependencies to show how failures in one layer propagate across systems.
- Improves machine learning accuracy by incorporating real-world outcomes into training data.
- Continuously updates incident libraries to enhance predictive precision.
Benefits of Historical Data Analysis in AiOps
- Faster identification of emerging outage risks.
- More accurate root cause predictions based on past events.
- Enables proactive detection of similar failure patterns across environments.
- Strengthens post-incident reviews with predictive insights for future prevention.
- Improves collaboration between IT operations, application teams, and DevOps.
Predictive Incident Management: Anticipating Problems Before They Escalate
Predictive AiOps doesnโt just forecast failures โ it changes how IT teams manage incidents entirely. Instead of waiting for alerts, AiOps predicts incidents in advance and creates preemptive incident records with context and recommended actions.
How Predictive Incident Management Works
- Detects early signs of service degradation through pattern analysis.
- Creates predictive incident tickets with preliminary root cause analysis.
- Links predicted incidents to associated infrastructure and services.
- Provides recommendations for preemptive fixes and escalation paths.
- **Prioritizes incidents based on business impact, service criticality, and risk probability.
Benefits of Predictive Incident Management
- Prevents minor issues from escalating into major outages.
- Reduces MTTD (Mean Time to Detect) and MTTR (Mean Time to Resolve).
- Gives IT teams advance warning, improving response coordination.
- Improves ITSM effectiveness by integrating predictive insights.
- Aligns incident management with proactive risk mitigation strategies.
Self-Healing IT: Combining Prediction with Automation
Prediction alone isnโt enough โ preventive action is critical. Leading Predictive AiOps platforms integrate with automation and orchestration tools to trigger self-healing actions based on predicted risks.
Key Elements of Self-Healing IT with Predictive AiOps
- Detect-Analyze-Act Loops that combine prediction, diagnosis, and action.
- Automated workflows for common issues like resource exhaustion, service crashes, and memory leaks.
- Human approval gates for high-risk changes, ensuring control over automation.
- Post-remediation reviews to feed learning back into predictive models.
- Pre-defined playbooks customized to different environments and applications.
Benefits of Self-Healing AiOps
- Prevents outages through preemptive remediation.
- Reduces manual workload for IT teams.
- Shortens incident recovery times with automated interventions.
- Continuously improves prevention accuracy through feedback loops.
- Increases system resilience in dynamic, fast-changing environments.
Business Impact of Staying Ahead of Outages with Predictive AiOps
Predictive AiOps isnโt just a technical tool โ itโs a business enabler. By predicting and preventing outages, AiOps helps protect revenue, maintain customer trust, and support innovation.
Business-Level Benefits of Predictive AiOps
- Reduces revenue loss caused by service disruptions.
- Protects brand reputation and customer satisfaction.
- Ensures compliance with regulatory uptime requirements.
- **Provides IT leaders with real-time risk visibility tied to business services.
- **Aligns IT performance with business goals and digital transformation initiatives.
How Business Leaders Benefit
- Predictable service levels for critical digital services.
- Data-backed assurance for product launches, seasonal spikes, and migrations.
- Improved collaboration between IT, DevOps, and business teams.
- Cost savings from fewer outages and more efficient infrastructure use.
- Stronger reporting and transparency into IT operational health.
Predictive AiOps โ A Critical Capability for Modern IT
In todayโs always-on digital world, staying ahead of outages is no longer optional โ itโs essential. Predictive AiOps provides the visibility, intelligence, and automation needed to proactively detect, predict, and prevent service disruptions before they impact users and revenue.
Key Takeaways
- Predictive AiOps transforms data into proactive insights.
- Combines anomaly detection, historical learning, and predictive modeling.
- Creates predictive incident records with preventive recommendations.
- Triggers automated remediation workflows for self-healing.
- Aligns IT operations with business continuity and user experience goals.
With Predictive AiOps in action, IT leaders gain the foresight and agility needed to ensure reliable, high-performance digital experiences โ every minute of every day.