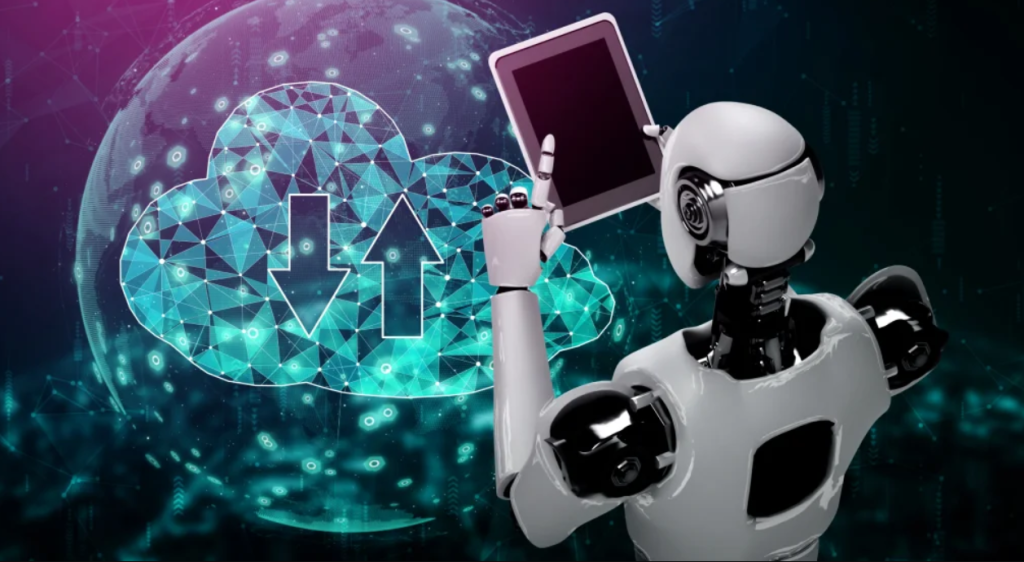
Introduction: From Reactive to Predictive — AiOps Redefining IT Operations
The rapid evolution of IT ecosystems has introduced unprecedented complexity into modern IT operations. Organizations today manage hybrid infrastructures, combining on-premise data centers, multi-cloud environments, containerized applications, microservices architectures, and edge deployments. This complexity brings with it a tsunami of data — logs, metrics, traces, configuration changes, and security events — all requiring real-time analysis to ensure seamless performance and system reliability.
Traditional IT operations practices rely heavily on reactive processes, where teams detect issues only after they disrupt critical business services. This after-the-fact approach is no longer viable in an era where every minute of downtime costs thousands of dollars, erodes customer trust, and disrupts digital transformation initiatives.
This is where AiOps (Artificial Intelligence for IT Operations) is reshaping the IT landscape. By using machine learning, predictive analytics, and automation, AiOps equips IT teams with the predictive power to forecast issues before they occur, enabling proactive mitigation, faster resolution, and optimized resource utilization. AiOps is no longer just a tool; it’s becoming the operational nerve center for future-proof IT ecosystems.
Why AiOps’ Predictive Power is a Game-Changer
- Shifts IT from reactive incident management to proactive risk prevention.
- Transforms raw operational data into real-time, actionable intelligence.
- Predicts system failures, performance degradation, and capacity bottlenecks.
- Improves IT efficiency, reduces costs, and enhances service reliability.
- Enables IT to align operations with business goals and user expectations.
Core Features Powering AiOps Predictive Capabilities
The predictive strength of AiOps stems from its ability to ingest, correlate, and analyze massive volumes of IT data in real time, applying advanced machine learning models to extract patterns and forecast future risks. AiOps combines multiple functionalities into a single predictive intelligence platform.
Key Features That Empower Predictive AiOps
- Multi-Layer Data Ingestion
- Ingests data from infrastructure, applications, networks, databases, cloud platforms, and security tools.
- Normalizes data across structured (metrics, logs) and unstructured (alerts, events) formats.
- Creates a unified data lake for cross-domain analysis.
- Dynamic Baseline Learning
- Continuously learns normal behavior across applications, servers, networks, and microservices.
- Updates baselines to reflect seasonal changes, traffic patterns, and system evolution.
- Adapts to new deployments, architectural changes, and dynamic workloads.
- Anomaly Detection and Event Correlation
- Detects subtle deviations before they breach hard thresholds.
- Correlates seemingly unrelated anomalies across dependent services and infrastructure layers.
- Predicts cascading failures based on anomaly chains and historical incident patterns.
- Predictive Analytics and Incident Forecasting
- Applies machine learning models to forecast failures, slowdowns, and bottlenecks.
- Assigns probability scores to predicted incidents and ranks them by business impact.
- Generates early warning alerts with recommended preventive actions.
- Proactive Recommendations and Automated Prevention
- Provides actionable insights — from scaling resources to adjusting configurations.
- Triggers automated playbooks for self-healing and risk mitigation.
- Learns from past outcomes to continuously improve prediction accuracy.
Trend 1: Convergence of Observability and Predictive AiOps
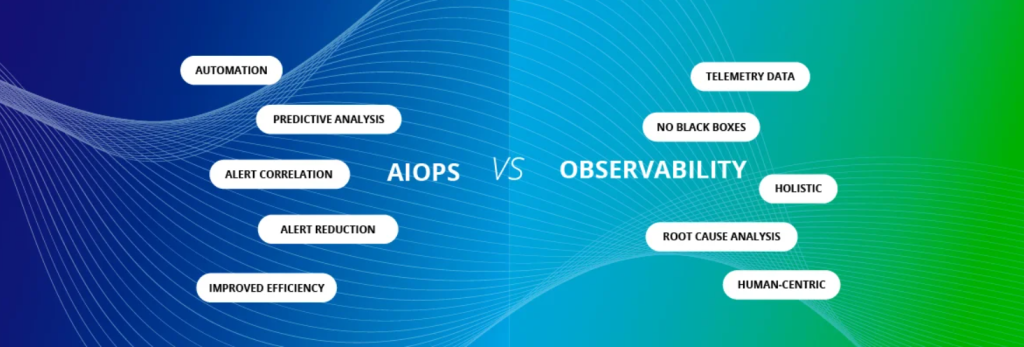
One of the foundational trends shaping AiOps is the merger between full-stack observability and predictive analytics. Organizations are moving away from isolated monitoring tools and towards integrated observability platforms that feed real-time performance data directly into AiOps engines.
Why Observability is Critical for Predictive AiOps
- Observability provides a 360-degree view of IT health across applications, infrastructure, and networks.
- Unified data from logs, metrics, traces, and events creates richer training data for AI models.
- Predictive models become more accurate when they can correlate anomalies across domains.
- Proactive issue detection becomes possible across the entire IT stack.
Benefits of Observability-Driven Predictive AiOps
- Complete visibility into system performance and emerging risks.
- Faster identification of root causes for predicted issues.
- Better correlation between IT events and business services.
- Improved collaboration across infrastructure, application, and DevOps teams.
- Higher accuracy in forecasting service-impacting incidents.
Trend 2: Predictive Incident Management Becomes Standard
Predictive incident management represents a seismic shift in ITSM processes. Instead of waiting for incidents to happen, AiOps can forecast potential incidents based on detected performance drifts, configuration changes, or anomaly patterns. This lets IT teams prepare and prevent, rather than react and repair.
Key Elements of Predictive Incident Management
- Predictive models identify incident precursors, such as abnormal latency spikes or config drift.
- Incidents are logged in advance with contextual data, root cause hypotheses, and probable impact.
- Predictive tickets integrate directly into ITSM platforms for pre-emptive action.
- Incident prevention workflows trigger automatically for well-understood risks.
Benefits of Predictive Incident Management
- Fewer critical incidents reach production systems.
- MTTD and MTTR reduced through proactive triage and diagnosis.
- Lower stress and improved productivity for IT operations teams.
- Reduces downtime and ensures consistent service reliability.
- Transforms ITSM from reactive ticketing to predictive risk management.
Trend 3: Capacity Forecasting and Cost Optimization with AiOps
Resource management is no longer just about provisioning servers — it’s about forecasting future capacity needs and aligning infrastructure with business demand. AiOps analyzes historical usage trends, seasonal demand spikes, and future growth projections to recommend capacity adjustments before shortages occur.
How AiOps Optimizes Capacity with Predictive Power
- Analyzes historical data to predict future demand trends.
- Identifies over-provisioned and under-utilized resources.
- Recommends scaling plans that balance cost and performance.
- Provides early alerts for potential capacity saturation points.
- Tracks costs to ensure spending aligns with business priorities.
Benefits of Predictive Capacity Management
- Prevents performance degradation due to capacity exhaustion.
- Optimizes cloud spending by rightsizing infrastructure.
- Improves financial forecasting for IT budgets.
- Ensures infrastructure scales with business growth.
- Reduces over-provisioning and wasted resources.
Trend 4: Self-Healing IT Becomes Proactive
Predictive power isn’t valuable without proactive action. Modern AiOps platforms don’t just predict failures — they trigger automated remediation actions to prevent them entirely.
Components of Self-Healing AiOps
- Predictive models detect high-likelihood failures.
- Automated workflows apply preventive actions (restart services, failover traffic).
- AI learns from remediation success to refine future recommendations.
- Human approvals added for high-risk actions.
Benefits of Proactive Self-Healing IT
- Prevents incidents without human intervention.
- Reduces unplanned outages and performance degradation.
- Improves system resilience and reliability.
- Speeds up incident recovery with automated actions.
- Creates a learning loop between prediction and prevention.
AiOps Predictive Power Shapes the Future of IT
As IT ecosystems grow more complex and dynamic, reactive operations simply can’t scale. Predictive AiOps empowers IT teams to anticipate, prevent, and optimize their systems — aligning technology performance with business priorities and customer expectations.
What Predictive AiOps Delivers
- Higher reliability through proactive incident prevention.
- Optimized infrastructure with predictive capacity planning.
- Faster response through pre-enriched incident data.
- Cost-efficient operations through smarter resource management.
- Strategic IT alignment with business and customer goals.