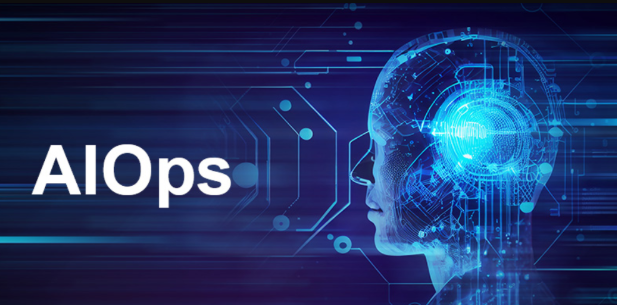
Introduction to AiOps Analytics: A New Era in IT Operations
In today’s rapidly evolving IT landscape, the sheer volume and complexity of data generated by IT systems make traditional monitoring techniques insufficient. To address this, AiOps (Artificial Intelligence for IT Operations) emerged, combining machine learning, automation, and data analytics to enhance operational efficiency and minimize disruptions. AiOps uses predictive analytics to not only detect and resolve issues but to anticipate and prevent potential incidents before they impact end-users. This shift towards predictive monitoring ensures a more proactive approach, which is essential for businesses that aim to stay competitive in an increasingly digital world.
By integrating AiOps into an organization’s IT operations, teams can gain unprecedented insights and control over their infrastructure, offering smarter, more efficient, and reliable monitoring capabilities.
Key Features of AiOps Analytics
AiOps Analytics comes with several distinctive features that are reshaping how organizations manage their IT operations. Here are some of the major features:
1. Real-Time Monitoring
AiOps provides continuous, real-time monitoring, which is crucial for detecting anomalies as they occur. This allows IT teams to respond immediately to incidents, reducing the impact on business operations.
- Instant anomaly detection: By analyzing data in real-time, AiOps identifies issues as soon as they appear.
- 24/7 system surveillance: AiOps offers continuous monitoring, ensuring that systems are under surveillance around the clock.
2. Predictive Analytics
AiOps leverages machine learning algorithms to predict future events based on historical data. This predictive capability allows organizations to address potential issues before they affect their systems or users.
- Trend analysis: AiOps analyzes data trends over time to forecast system behavior and identify potential failures.
- Proactive incident resolution: By predicting disruptions, AiOps enables teams to take action ahead of time, minimizing downtime.
3. Root Cause Analysis (RCA)
When issues do occur, AiOps enables teams to quickly perform root cause analysis, enabling a faster resolution.
- Fast problem identification: AiOps can instantly detect the underlying cause of system failures.
- Data-driven insights: RCA uses vast amounts of historical data to pinpoint the exact issue, improving troubleshooting efficiency.
4. Automated Remediation
One of the most powerful aspects of AiOps is its ability to automate remediation efforts, allowing systems to self-correct without manual intervention.
- Automated responses: AiOps can automatically resolve common issues such as server restarts or resource allocation.
- Reduced manual workload: This reduces the burden on IT staff, allowing them to focus on more strategic tasks.
5. Scalability
AiOps tools are designed to scale with the growth of the organization, ensuring that they remain effective even as the IT environment becomes more complex.
- Adaptability: AiOps can monitor a wide range of systems, whether cloud-based, on-premise, or hybrid.
- Efficient resource allocation: AiOps can adjust monitoring thresholds and scale resources according to demand, optimizing system performance.
Benefits of AiOps Analytics for Smarter Monitoring
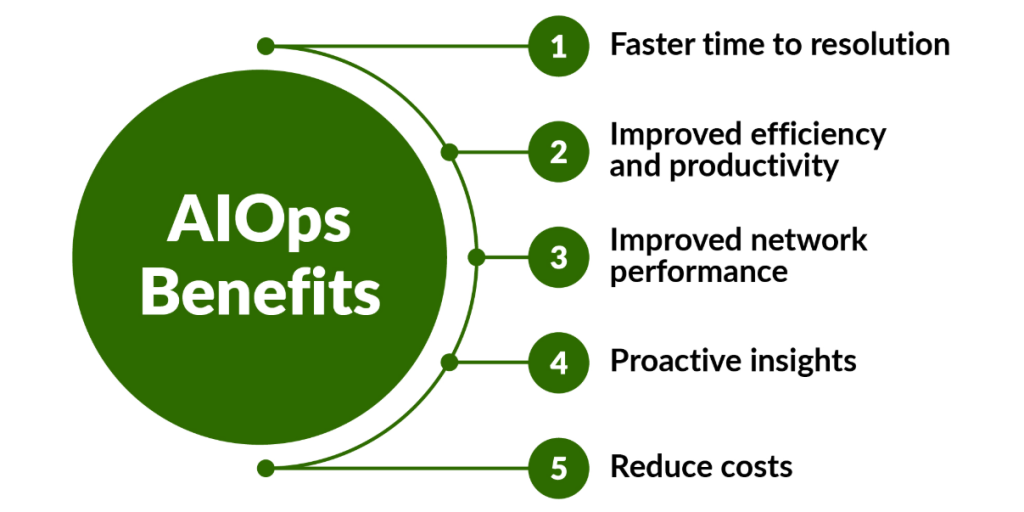
The advantages of implementing AiOps Analytics are numerous and far-reaching. Here are the key benefits:
1. Proactive Problem Management
Rather than reacting to problems as they arise, AiOps enables IT teams to anticipate potential disruptions and take action to prevent them.
- Minimized downtime: Predicting issues before they occur means businesses can avoid downtime, leading to improved productivity.
- Enhanced service reliability: AiOps ensures that systems run smoothly by addressing issues before they escalate.
2. Cost Savings
With fewer outages, more efficient resource allocation, and reduced manual intervention, AiOps can significantly lower operational costs.
- Decreased operational expenses: By automating incident resolution and reducing downtime, AiOps helps lower the total cost of IT operations.
- Optimized resource usage: AiOps ensures that resources are allocated efficiently, reducing waste and unnecessary expenses.
3. Improved Decision-Making
AiOps empowers IT teams to make better, data-driven decisions, improving the overall strategic management of IT systems.
- Actionable insights: AiOps doesn’t just provide data but interprets it, offering actionable recommendations.
- Faster decision-making: With real-time analytics and predictive insights, decision-makers can act swiftly and confidently.
4. Increased Operational Efficiency
AiOps streamlines IT operations by automating routine tasks and improving the speed and accuracy of incident resolution.
- Faster response times: Automation and predictive analytics ensure that issues are dealt with much quicker than with traditional monitoring.
- Operational optimization: AiOps improves the efficiency of daily operations by providing more accurate data and automating repetitive processes.
5. Enhanced System Reliability and Stability
By predicting and preventing potential system failures, AiOps ensures that your IT infrastructure remains stable and reliable.
- Reduced failure rates: AiOps reduces the frequency and severity of system failures by predicting and preventing them in advance.
- Increased uptime: With fewer interruptions and quicker resolutions, AiOps ensures a more stable IT environment.
AiOps Analytics vs. Traditional IT Monitoring
While traditional IT monitoring methods have served organizations well for many years, AiOps introduces a major shift in how IT operations are handled. Here’s how AiOps differs from traditional approaches:
1. Proactivity vs. Reactivity
Traditional IT monitoring focuses largely on reactive approaches—responding to incidents after they happen. AiOps, however, focuses on preventing issues before they occur, providing proactive insights and predictions.
- Traditional monitoring: Primarily responds to issues that have already occurred, resulting in longer recovery times.
- AiOps: Anticipates problems and takes action before they cause disruptions, resulting in quicker resolution times and less downtime.
2. Human-Driven vs. Automated Processes
Traditional monitoring tools often require human intervention to detect and resolve issues, leading to potential delays. AiOps integrates automation to handle issues without human involvement, which enhances speed and reduces error.
- Traditional monitoring: Often requires manual configuration and monitoring by IT staff.
- AiOps: Automates troubleshooting, remediation, and predictive analysis, reducing the need for human involvement in routine tasks.
3. Static Monitoring vs. Dynamic Adaptation
Traditional monitoring tools often rely on fixed thresholds for alerts and notifications, which can lead to either missed issues or an overload of false alarms. AiOps, by contrast, dynamically adapts its monitoring thresholds and responses based on the system’s current state and predicted future behavior.
- Traditional monitoring: Fixed thresholds can lead to false positives or undetected issues.
- AiOps: Learns from past data to adjust thresholds dynamically, providing more accurate insights.
Challenges in Implementing AiOps Analytics
While AiOps offers substantial benefits, it also presents challenges that organizations must address during implementation:
1. Data Quality and Consistency
AiOps relies heavily on data to make predictions and recommendations. Inconsistent or poor-quality data can negatively impact the effectiveness of AiOps.
- Data cleansing: Organizations must invest in maintaining high-quality, consistent data to fully benefit from AiOps.
- Data integration: Ensuring that AiOps platforms can access and integrate data from various sources is critical to success.
2. Complexity of Integration
Integrating AiOps tools with existing IT infrastructure can be complex, especially if the organization uses legacy systems.
- System compatibility: AiOps must be compatible with all parts of the existing infrastructure for smooth integration.
- Customization: Some systems may require customization to work with AiOps, which can be time-consuming.
3. Skillset Requirements
AiOps tools often require specialized knowledge to set up, operate, and interpret the data. IT teams may need additional training or new skill sets to maximize the potential of AiOps solutions.
- Training programs: IT staff must be adequately trained to understand AiOps platforms and their advanced functionalities.
- Specialized roles: New roles, such as data scientists and AI specialists, may be required to manage AiOps effectively.
The Future of AiOps Analytics
As businesses increasingly adopt digital transformation strategies, the role of AiOps will become even more vital. Here are some predictions for the future of AiOps:
1. Wider Adoption of AI and Machine Learning
As AI and machine learning technologies continue to evolve, they will become even more integrated into AiOps solutions, making them more powerful and accurate.
- More accurate predictions: Future AiOps solutions will use even more advanced algorithms to predict problems with greater accuracy.
- Enhanced machine learning: The learning capabilities of AiOps will improve, enabling the system to evolve continuously.
2. Edge Computing and IoT Integration
With the rise of edge computing and IoT devices, AiOps will extend beyond centralized systems to provide predictive monitoring at the edge.
- Decentralized monitoring: AiOps will enable monitoring and predictions at the edge, helping to manage data from IoT devices.
- Real-time edge analytics: AiOps will process data from edge devices in real-time, improving responsiveness.
3. AI-Driven Autonomous IT Operations
The ultimate goal of AiOps is to create autonomous IT systems that can self-manage based on predictive insights and machine learning algorithms.
- Self-healing systems: AiOps will lead to fully autonomous systems capable of self-healing and optimization without human intervention.
- Zero-touch IT operations: Over time, AiOps will manage entire IT operations with minimal human oversight, reducing the workload on IT teams.