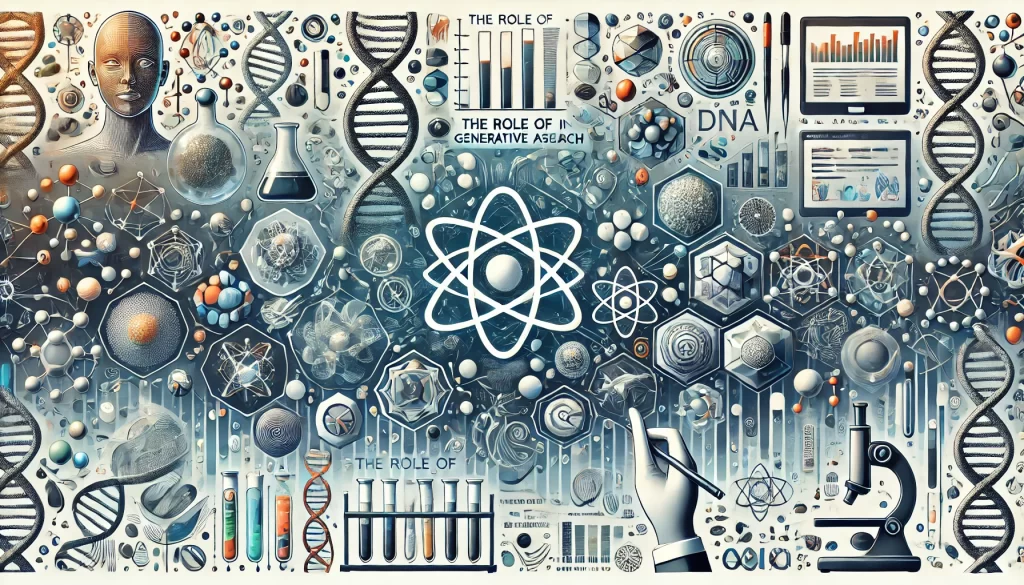
Generative AI has emerged as a transformative technology in scientific research, helping researchers explore complex data, simulate experiments, generate hypotheses, and accelerate breakthroughs. Unlike traditional AI, which primarily analyzes and predicts based on existing data, generative AI can create new data, ideas, and insights, offering unprecedented opportunities to advance knowledge across diverse scientific disciplines. Here, we explore the role of generative AI in scientific research, examining its applications, benefits, and impact on how science is conducted in fields ranging from drug discovery to climate science.
Accelerating Drug Discovery and Development
One of the most promising applications of generative AI in scientific research is in the field of drug discovery, where it helps researchers identify new compounds, simulate molecular interactions, and optimize drug candidates.
- Applications:
- Molecule Generation: Generative AI models, such as variational autoencoders (VAEs) and generative adversarial networks (GANs), generate novel molecular structures that may have therapeutic properties. These models can design molecules with specific characteristics, enabling researchers to discover potential drugs more efficiently.
- Predicting Drug-Protein Interactions: Generative AI models can simulate how potential drugs interact with proteins in the human body, helping researchers predict efficacy and potential side effects.
- Optimization of Drug Candidates: AI can refine existing molecules to improve properties like bioavailability, reducing toxicity while enhancing effectiveness, and speeding up the process of moving from lab tests to clinical trials.
- Impact: Generative AI shortens the drug discovery process from years to months, reducing costs and making it easier to develop targeted therapies. This acceleration is crucial for rapidly addressing urgent health crises, such as finding treatments for new viral outbreaks or rare diseases.
Advancing Material Science
Generative AI is transforming materials science by enabling researchers to design new materials with specific properties, predict the behavior of compounds, and simulate manufacturing processes.
- Applications:
- Designing Advanced Materials: Generative AI can create materials optimized for strength, flexibility, conductivity, or other properties, which is valuable for fields like electronics, aerospace, and renewable energy.
- Predicting Material Properties: By analyzing existing data, generative AI can predict how a new material might behave under different conditions, helping researchers find ideal materials faster.
- Optimizing Manufacturing Processes: Generative AI can simulate the effects of different manufacturing techniques, identifying the optimal processes to produce high-quality materials efficiently.
- Impact: Generative AI in materials science accelerates the development of sustainable materials, lightweight composites, and superconductors, potentially leading to more energy-efficient technologies and significant advancements in multiple industries.
Simulating Climate and Environmental Models
Climate science involves modeling complex systems with numerous interacting variables, making it an ideal field for generative AI. By creating detailed simulations, AI can help scientists understand and predict the effects of climate change with greater accuracy.
- Applications:
- Climate Modeling: Generative AI helps create and refine models that predict the impact of various factors (such as greenhouse gases and deforestation) on global temperatures, weather patterns, and sea levels.
- Simulating Environmental Scenarios: AI models can simulate potential environmental interventions, such as reforestation or emission reduction, to predict their long-term effects.
- Biodiversity and Ecosystem Research: Generative models can simulate ecosystems, helping scientists understand how changes in the environment might impact biodiversity and species interactions.
- Impact: Generative AI provides a more comprehensive understanding of climate dynamics, enabling policymakers to make informed decisions. It allows scientists to test hypotheses quickly and evaluate the effectiveness of mitigation strategies, potentially guiding efforts to combat climate change.
Enhancing Genomics and Precision Medicine
In genomics, generative AI supports precision medicine by helping researchers analyze genetic data, model protein structures, and explore personalized treatment options based on individual genetic profiles.
- Applications:
- Genetic Sequence Generation: AI models generate synthetic genetic sequences that simulate different gene variations, aiding in the study of gene functions and interactions.
- Protein Structure Prediction: Generative AI predicts protein folding patterns, which is critical for understanding diseases and developing targeted treatments.
- Personalized Treatment Planning: By analyzing a patient’s genetic data, generative AI can recommend personalized therapies, such as identifying which cancer treatments may be most effective based on specific genetic markers.
- Impact: Generative AI in genomics accelerates advancements in personalized medicine, allowing treatments to be tailored to individuals. This leads to more effective therapies with fewer side effects, improving patient outcomes and pushing medicine towards more preventive, targeted approaches.
Optimizing Energy Solutions and Sustainability
Generative AI is playing a vital role in the development of sustainable energy solutions. From optimizing renewable energy sources to advancing battery technology, AI-driven research can support the transition to greener energy.
- Applications:
- Designing Efficient Solar Cells: Generative models simulate new materials and configurations for solar panels, maximizing energy capture and improving efficiency.
- Improving Battery Performance: AI generates new battery materials that enhance capacity, durability, and charge cycles, supporting advancements in electric vehicles and renewable energy storage.
- Energy Grid Optimization: Generative AI models help optimize power grids by simulating load distributions and predicting energy demand, improving efficiency and reducing waste.
- Impact: AI-driven innovations in energy storage, generation, and distribution support a more sustainable future. By accelerating research, generative AI helps bring environmentally friendly technologies to market faster, addressing global energy needs more sustainably.
Augmenting Astronomy and Space Exploration
Generative AI is helping astronomers analyze large datasets from telescopes, simulate cosmic events, and even plan space missions. With its ability to generate models and predictions, AI is unlocking new insights into the universe.
- Applications:
- Simulating Cosmic Events: Generative AI models simulate events like supernovae, black hole formations, and galaxy evolution, providing insights into how these phenomena occur.
- Analyzing Telescope Data: AI algorithms can analyze massive datasets from space telescopes, identifying patterns and anomalies that human researchers might miss.
- Supporting Mission Planning: AI helps design spacecraft, simulate trajectories, and predict challenges for deep space missions, making exploration safer and more feasible.
- Impact: Generative AI accelerates discoveries in astrophysics and helps plan ambitious space missions. By simulating complex astronomical events and providing new ways to analyze data, AI expands humanity’s understanding of the cosmos.
Revolutionizing Computational Chemistry
In computational chemistry, generative AI supports the design and simulation of chemical compounds and reactions, enabling researchers to explore new frontiers in chemical synthesis and catalysis.
- Applications:
- Predicting Chemical Reactions: AI models simulate how different molecules will react under various conditions, helping chemists predict outcomes without needing physical experimentation.
- Catalyst Design: Generative AI identifies catalysts that can accelerate chemical reactions, making industrial processes more efficient and environmentally friendly.
- Synthesizing New Compounds: AI can generate novel molecular structures with specific properties, such as stability, solubility, or reactivity, supporting the development of new materials and pharmaceuticals.
- Impact: Generative AI in computational chemistry accelerates the discovery of new materials and chemicals, enabling safer, more sustainable industrial practices and helping chemists innovate more effectively.
Advancing Neuroscience and Brain Research
Generative AI is contributing to neuroscience by helping researchers simulate brain functions, analyze neural patterns, and generate models of brain disorders, supporting efforts to understand the complexities of the human brain.
- Applications:
- Modeling Neural Pathways: Generative models simulate how neurons interact, helping scientists understand complex brain functions and disorders.
- Brain Mapping and Imaging: AI-driven techniques enhance brain imaging, generating detailed maps of brain regions and neural activity.
- Simulating Disease Progression: AI models simulate how brain disorders, such as Alzheimer’s and Parkinson’s, progress, supporting the development of targeted treatments.
- Impact: Generative AI in neuroscience accelerates understanding of brain functions and disorders, potentially leading to breakthrough treatments for neurological conditions and improved mental health therapies.
Augmenting Theoretical Research with Hypothesis Generation
Generative AI has the potential to generate and test scientific hypotheses, acting as a virtual research assistant that explores possibilities, validates theories, and proposes experiments.
- Applications:
- Data Exploration: AI models analyze complex datasets to identify patterns, correlations, and anomalies, suggesting potential hypotheses.
- Experiment Simulation: Generative AI simulates experiments based on theoretical assumptions, enabling researchers to test ideas without costly lab work.
- Hypothesis Testing: By evaluating scientific literature, AI can propose hypotheses that align with existing knowledge while identifying gaps for further exploration.
- Impact: AI-driven hypothesis generation enables researchers to explore scientific questions at a faster pace, reducing trial-and-error and focusing on promising avenues for experimentation.