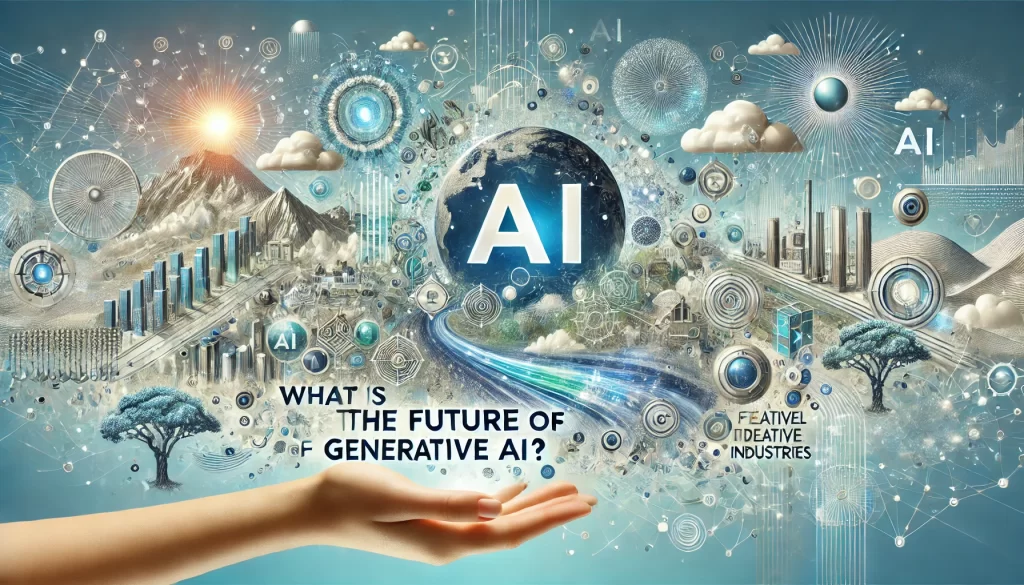
The future of generative AI holds significant potential across various fields, promising advancements that could further integrate this technology into everyday life and professional applications. As models become more sophisticated, generative AI is expected to create even more realistic and nuanced content, which could enhance virtual environments, personalized content creation, and creative tools in industries like film, design, and gaming. AI is also likely to play a greater role in fields such as healthcare and education, where it can simulate complex scenarios for training or generate customized learning materials. Improvements in model interpretability and control could make AI-generated content more predictable and manageable, reducing some of the risks associated with current models.
Improving Realism and Quality
One of the main goals for the future of generative AI is to produce even more realistic and high-quality outputs, whether in text, images, video, or audio. Advances in model architecture and training techniques will allow generative AI to generate content that is nearly indistinguishable from human-created content.
- Examples: Ultra-realistic images and videos for movies and virtual reality, highly sophisticated chatbots, and lifelike audio for realistic virtual assistants.
- Impact: Improved realism will open new opportunities in industries like entertainment, advertising, and virtual experiences, allowing creators to push the boundaries of what’s possible.
Multimodal Generative AI
The future of generative AI will increasingly involve multimodal models that can handle and combine multiple types of data—text, image, audio, and video—within a single model. This will allow for the seamless creation of content that incorporates multiple media, enhancing applications in areas like storytelling, virtual reality, and interactive experiences.
- Examples: AI models that can create entire scenes with visuals, narration, sound effects, and background music based on a simple text prompt.
- Impact: Multimodal AI will make it easier for creators to produce rich, immersive experiences for entertainment, education, marketing, and beyond.
Personalization and Hyper-Customization
Generative AI’s ability to create personalized content on a large scale is set to grow significantly, helping businesses, educators, and creators deliver tailored experiences. Future AI models will be able to generate highly customized outputs for individual users, making recommendations, interactions, and content more relevant and engaging.
- Examples: Personalized virtual learning modules, custom advertising, and tailored healthcare plans based on individual preferences and history.
- Impact: Hyper-customization will enhance user engagement, satisfaction, and outcomes across multiple sectors, from e-commerce and education to healthcare and entertainment.
Reducing Computational Demands
Current generative AI models often require immense computational resources, but the future will likely bring more efficient models that maintain high performance while reducing computational demands. Researchers are developing new training techniques, architectures, and optimization methods to create smaller, faster, and more energy-efficient models.
- Examples: Lightweight models that can run on mobile devices or embedded systems without compromising quality.
- Impact: Reduced resource demands will make generative AI more accessible and sustainable, enabling smaller organizations to leverage powerful AI and reduce the environmental footprint of AI.
Real-Time and Interactive Generative AI
As generative AI improves in speed and efficiency, we’ll likely see more real-time applications where AI responds instantly to user input. Real-time generative AI will allow for interactive experiences, such as dynamic game environments, live language translation, and adaptive content in virtual reality.
- Examples: Video games that adjust environments, characters, or storylines based on player actions; AI-driven storytelling that adapts to reader choices in real-time.
- Impact: Real-time generative AI will create a new level of engagement and immersion, especially in entertainment, gaming, and education, allowing users to actively shape their experiences.
Generative AI in Creative Collaboration
Generative AI has already become a powerful tool for creatives, but the future will see AI becoming an even more integral part of the creative process. AI will act as a collaborative partner, assisting artists, writers, and designers with ideation, execution, and refinement, freeing them to focus on higher-level creativity.
- Examples: AI tools that help writers brainstorm plot twists, musicians experimenting with unique sounds, or visual artists exploring new styles.
- Impact: AI as a creative collaborator will amplify human creativity, expanding artistic possibilities and allowing creators to bring new visions to life.
Enhanced Data Privacy and Ethics in Generative AI
The future of generative AI will prioritize ethical considerations, focusing on reducing bias, protecting privacy, and ensuring accountability. As public awareness of AI ethics grows, developers and organizations must adopt practices and regulations that address these concerns, creating transparent and responsible generative models.
- Examples: AI tools that allow users to control how their data is used, mechanisms to flag and minimize bias, and regulatory frameworks for ethical AI use.
- Impact: Focusing on ethics and privacy will build trust in generative AI technologies, encouraging wider adoption and responsible use across industries.
Generative AI in Scientific Discovery and Problem Solving
Generative AI will increasingly be used to address complex problems, from drug discovery and climate change to space exploration. By simulating scenarios, generating hypotheses, or designing experiments, AI can assist scientists and researchers in tackling some of humanity’s most challenging issues.
- Examples: AI models that propose new molecular structures for drug development, simulate climate solutions, or generate insights in material science.
- Impact: Generative AI in scientific research will accelerate breakthroughs, leading to new solutions in medicine, environmental science, and technology.
Democratization of Generative AI
As generative AI models become more efficient and accessible, they will no longer be exclusive to large tech companies. The democratization of AI will allow smaller businesses, independent creators, and individuals to use AI tools, leading to more diverse applications, ideas, and innovations.
- Examples: Open-source generative AI tools, user-friendly interfaces for non-experts, and platforms that enable creators to access powerful AI models.
- Impact: Democratized generative AI will foster innovation from a broader range of voices, increasing creativity and diversity in AI-driven content and applications.
Hybrid Models and Human-AI Collaboration
The future of generative AI will likely involve hybrid models that combine generative AI with other forms of machine learning, such as reinforcement learning and supervised learning, for more nuanced outputs. These models will enable enhanced human-AI collaboration, where AI can assist with complex decision-making, analysis, and interpretation.
- Examples: AI-assisted diagnostic tools that provide suggestions for doctors, content editing platforms that enhance writer input, or design platforms that adapt based on feedback.
- Impact: Hybrid models and collaboration with AI will amplify human expertise in various domains, combining human intuition with AI’s computational power to solve complex problems.