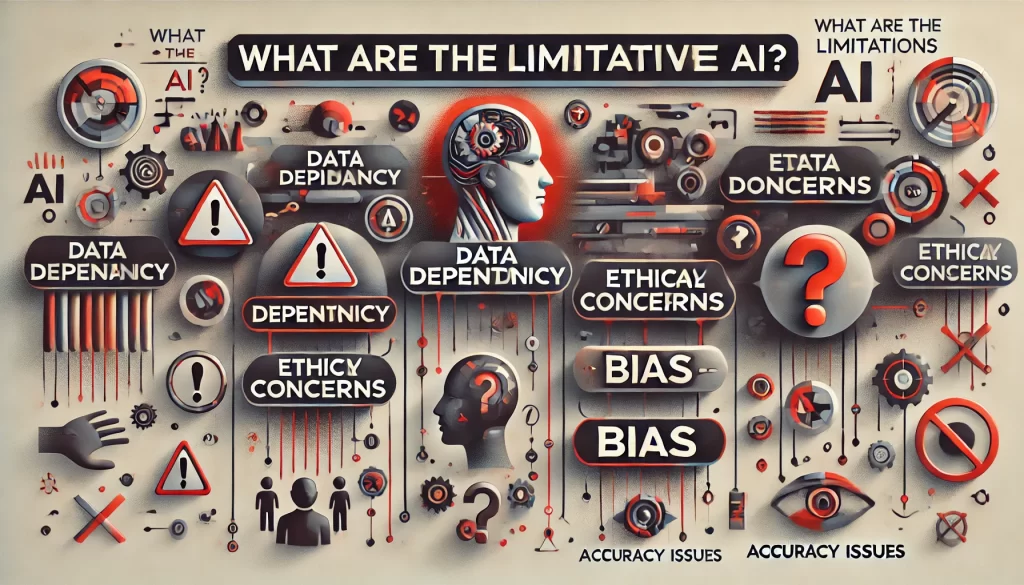
Generative AI, while powerful, has several limitations that impact its effectiveness and reliability. One key limitation is its reliance on large datasets for training; models trained on biased or unrepresentative data can produce outputs that reflect these biases, leading to skewed or inappropriate results. Another limitation is the lack of true understandingโAI generates content based on patterns rather than genuine comprehension, so it can produce outputs that appear plausible but are factually incorrect or nonsensical. Generative AI models are also computationally intensive, requiring significant processing power and resources, which can be costly and limit accessibility. Moreover, controlling the specific output of generative AI can be challenging; fine-tuning results often requires trial and error, and the models can sometimes produce unpredictable or unwanted content. Lastly, these models lack ethical judgment, meaning they can be easily misused to create harmful or misleading content, which requires human oversight to manage responsibly. Addressing these limitations involves careful model training, responsible data handling, and continuous improvement in AI interpretability and control mechanisms.
Dependence on Large, High-Quality Datasets
Generative AI models, especially powerful ones like GPT or DALL-E, rely heavily on vast amounts of high-quality, diverse data. These models learn patterns and relationships from the data theyโre trained on, which often requires millions of examples to yield accurate, varied outputs. However, gathering such data can be challenging, particularly in specialized or sensitive fields.
- Implications: Limited or low-quality data can lead to poor model performance, overfitting, or bias. Models may struggle with specialized applications, such as medical imaging, where data availability is often restricted due to privacy laws.
- Example: An AI model trained with insufficient data in a specific language or dialect may fail to generate accurate or meaningful content for that language group.
Bias and Fairness Issues
Generative AI models learn from historical data, which often contains embedded biases related to gender, race, or socioeconomic status. If these biases are not mitigated, AI models can perpetuate or even amplify them, leading to biased or unfair outputs. This is a critical concern, especially for applications in content creation, hiring, or customer service.
- Implications: Biased outputs can reinforce harmful stereotypes, limit inclusivity, and erode trust in AI systems.
- Example: A generative text model may produce gender-biased job descriptions if itโs trained on a dataset where certain roles are predominantly associated with specific genders.
High Computational and Energy Costs
Training and deploying large generative models requires significant computational power and energy. Training models like GPT-4 or DALL-E involve millions of parameters and large data sets, which translates to high energy consumption and substantial hardware costs. This limits the accessibility of generative AI to larger organizations and has a notable environmental impact.
- Implications: High resource demands restrict generative AIโs availability, contribute to carbon emissions, and pose sustainability concerns.
- Example: Training a large model like GPT-3 requires the equivalent energy consumption of multiple households over a year, raising concerns about AIโs environmental footprint.
Lack of True Understanding or Intent
Generative AI models, despite their sophisticated outputs, do not possess true understanding, intent, or reasoning. They generate content based on learned patterns, without comprehending the meaning behind their responses. This limitation can lead to irrelevant, incorrect, or nonsensical outputs, particularly when responding to complex questions or ethical issues.
- Implications: AI-generated content may lack depth, relevance, or appropriateness in sensitive contexts.
- Example: An AI chatbot responding to a customer complaint may produce empathetic language but miss the nuance or specific context of the complaint, leading to customer frustration.
Inability to Ensure Accuracy or Verify Information
Generative AI lacks built-in mechanisms to fact-check or verify the information it produces, which is a significant limitation in applications that require factual accuracy. Without external validation, these models may confidently generate plausible but incorrect information, creating risks in fields like healthcare, education, and journalism.
- Implications: Incorrect outputs can spread misinformation, erode trust, and potentially cause harm in critical areas.
- Example: A language model generating medical advice could produce incorrect information about treatments, potentially misleading users if not verified by experts.
Intellectual Property and Copyright Issues
Generative AI models are typically trained on massive datasets sourced from the internet, which often contain copyrighted material. This raises questions about the ownership of AI-generated content and the rights of the original creators whose work was used for training. Copyright infringement concerns limit generative AIโs commercial applicability and create ethical dilemmas.
- Implications: AI-generated content may infringe on copyrights, leading to legal disputes and impacting creatorsโ rights.
- Example: An AI-generated artwork that closely resembles a copyrighted piece may face legal challenges, complicating its commercial use.
Challenges in Consistency and Quality Control
Generative AI models can be unpredictable in quality, producing coherent, high-quality responses in some instances and low-quality or irrelevant outputs in others. This inconsistency can make it difficult to rely on AI-generated content for professional or public-facing applications where consistency is critical.
- Implications: Inconsistent quality reduces reliability and often requires human oversight for quality assurance.
- Example: An AI that generates customer support responses may provide helpful answers for common queries but struggle with unique or complex issues, requiring human intervention to ensure satisfaction.
Dependency and Risk of Reduced Human Creativity
Generative AIโs capabilities can lead to an over-reliance on AI for creative work, potentially stifling human originality. While AI can be a powerful tool for ideation, relying too heavily on it may discourage individuals from developing unique ideas or reduce diversity in creative fields.
- Implications: Over-reliance on AI could result in homogenized content, limiting the diversity of perspectives in creative industries.
- Example: A marketing team that consistently uses AI for ad copy may miss out on innovative ideas that come from human brainstorming and cultural insights.
Ethical Concerns and Misinformation
Generative AI can create content that is intentionally misleading, such as deepfakes or fake news. When AI generates realistic but false images, audio, or video, it poses significant risks for misinformation, fraud, and public manipulation, impacting trust in media and digital content.
- Implications: AI-generated misinformation can harm public opinion, damage reputations, and influence events like elections or court cases.
- Example: Deepfake technology can be used to fabricate videos of public figures, spreading false statements that could influence public opinion or defame individuals.