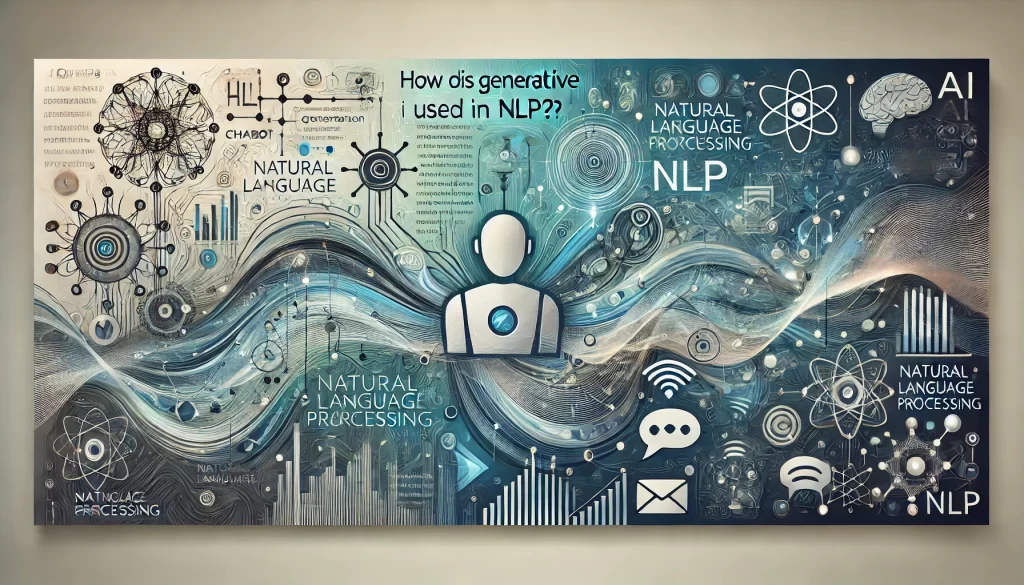
Generative AI has brought groundbreaking advancements to the field of natural language processing (NLP), empowering machines to understand, generate, and respond to human language in increasingly sophisticated ways. By leveraging powerful AI models, NLP systems can now produce human-like text, translate languages, summarize content, answer questions, and even generate creative writing. Hereโs an in-depth look at how generative AI is transforming NLP and reshaping applications across industries.
Text Generation and Content Creation
One of the most impactful uses of generative AI in NLP is text generation, where models produce coherent, contextually relevant text based on prompts. Large language models like GPT-3 and GPT-4 from OpenAI are trained on vast datasets of text, enabling them to generate essays, stories, product descriptions, and more with minimal human input. This technology is widely used across content creation industries, from marketing to journalism and even creative writing.
- Example Applications: Blog posts, news articles, product descriptions, ad copy, and social media posts.
- Benefits: Saves time for writers, improves content variety, and enables personalization for different audiences.
Conversational AI and Chatbots
Generative AI has significantly improved the capabilities of conversational AI, powering chatbots and virtual assistants that can interact with users naturally. By understanding the context and nuances in user queries, generative models allow chatbots to provide relevant responses, resolve issues, and engage users in human-like conversations. These AI-powered chatbots are used in customer service, mental health support, personal assistance, and more.
- Example Applications: Customer support chatbots, virtual personal assistants (e.g., Siri, Alexa), and mental health support bots.
- Benefits: Reduces response times, offers 24/7 support, and personalizes customer experiences.
Language Translation and Multilingual Content
Generative AI has revolutionized language translation by enabling more accurate and nuanced translations between multiple languages. Advanced models like OpenAIโs GPT and Googleโs Multilingual Neural Machine Translation (NMT) are capable of understanding idiomatic expressions, cultural context, and linguistic subtleties, allowing for translations that feel more natural. This technology has made it easier for businesses to communicate globally and for content to be accessible in multiple languages.
- Example Applications: Real-time translation in apps, multilingual content generation, and cross-cultural communication.
- Benefits: Reduces language barriers, improves communication, and makes information accessible to a wider audience.
Summarization of Text and Information Extraction
Generative AI models are also skilled at summarizing long texts, capturing the main ideas, and condensing information into shorter, digestible formats. This is particularly useful for news agencies, research institutions, and content-heavy organizations that need to process large volumes of text efficiently. NLP models can summarize articles, research papers, and even entire books, extracting key points while preserving the original meaning.
- Example Applications: News summarization, academic research summaries, document summarization, and report generation.
- Benefits: Saves time, improves information accessibility, and helps readers focus on essential insights.
Question Answering and Knowledge Retrieval
Generative AI has made strides in question-answering tasks, where models respond to user queries with precise and contextually relevant answers. By understanding questions and retrieving information from a knowledge base, generative AI can answer complex questions based on its training data or external databases. This is useful in customer service, educational platforms, and any context where users seek quick and accurate information.
- Example Applications: Virtual tutoring, FAQ automation, and interactive customer support.
- Benefits: Improves accessibility to information, enables interactive learning, and enhances user satisfaction by providing immediate responses.
Sentiment Analysis and Emotional Understanding
Generative AI models can analyze text to determine the underlying sentiment, such as whether a piece of writing is positive, negative, or neutral. This capability is crucial in customer feedback analysis, social media monitoring, and brand management, where businesses need to understand how customers feel about their products or services. Generative models go beyond basic sentiment analysis by generating empathetic responses, helping brands respond to customers in a more human-like, understanding manner.
- Example Applications: Social media monitoring, customer feedback analysis, and brand reputation management.
- Benefits: Enhances customer engagement, provides insights into customer satisfaction, and helps companies manage their brand image effectively.
Creative Writing and Ideation
Generative AI models like GPT-4 are increasingly being used for creative writing, ideation, and brainstorming, making them valuable tools for writers, marketers, and content creators. AI models can generate storylines, dialogue, plot ideas, and even poetry, providing inspiration for creative professionals and accelerating the content creation process.
- Example Applications: Scriptwriting, novel writing, poetry generation, and idea generation for advertising campaigns.
- Benefits: Enhances creative workflows, provides inspiration, and saves time in brainstorming and drafting.
Key Benefits of Generative AI in NLP
Generative AI in NLP offers several overarching benefits, including:
- Scalability: AI-generated text can be scaled across multiple platforms, enabling large-scale content creation and customer interactions.
- Efficiency: Automation of repetitive tasks like data extraction, summarization, and basic customer support improves efficiency for businesses.
- Personalization: By tailoring responses and content to individual users, generative AI enhances customer engagement and satisfaction.
- Global Accessibility: Language translation and multilingual capabilities make content and communication more accessible to a global audience.
Challenges and Ethical Considerations
While generative AI in NLP has transformative potential, it comes with challenges:
- Bias: Generative models may reproduce biases present in their training data, leading to biased outputs.
- Misuse: AI-generated text can be used to create misinformation or spam, raising ethical concerns.
- Privacy: Handling sensitive data in applications like chatbots requires careful consideration of privacy and security.