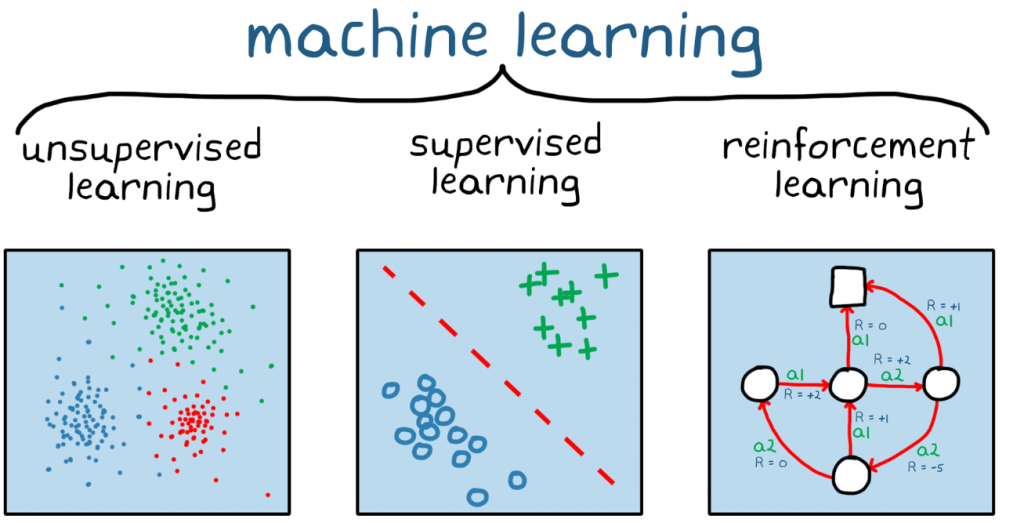
Machine learning is a broad field with various approaches to solving problems. Three primary types of machine learning stand out: Supervised, Unsupervised, and Reinforcement Learning. Each method has its unique characteristics, strengths, and applications.
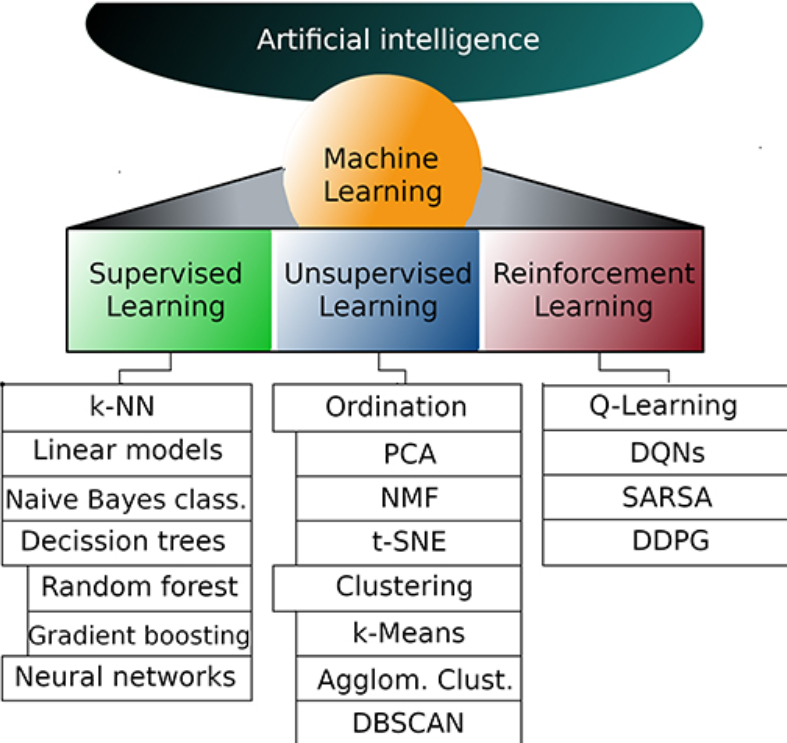
Supervised Learning
- Definition: In supervised learning, an algorithm learns from labeled data. This means the data used to train the algorithm includes desired outputs or correct answers.
- Process: The algorithm learns to map input data to output labels by identifying patterns and relationships between them.
- Common Algorithms: Linear Regression, Logistic Regression, Decision Trees, Random Forests, Support Vector Machines.
- Applications: Image recognition, spam filtering, fraud detection, medical diagnosis, customer churn prediction.
Unsupervised Learning
- Definition: Unsupervised learning deals with unlabeled data. The algorithm finds patterns, structures, or relationships within the data without any prior knowledge.
- Process: The algorithm explores the data to discover hidden patterns and group similar data points together.
- Common Algorithms: K-means Clustering, Hierarchical Clustering, Principal Component Analysis (PCA), t-Distributed Stochastic Neighbor Embedding (t-SNE). Â 1. dataguy.in dataguy.in
- Applications: Customer segmentation, image and document clustering, anomaly detection, feature engineering, recommendation systems.
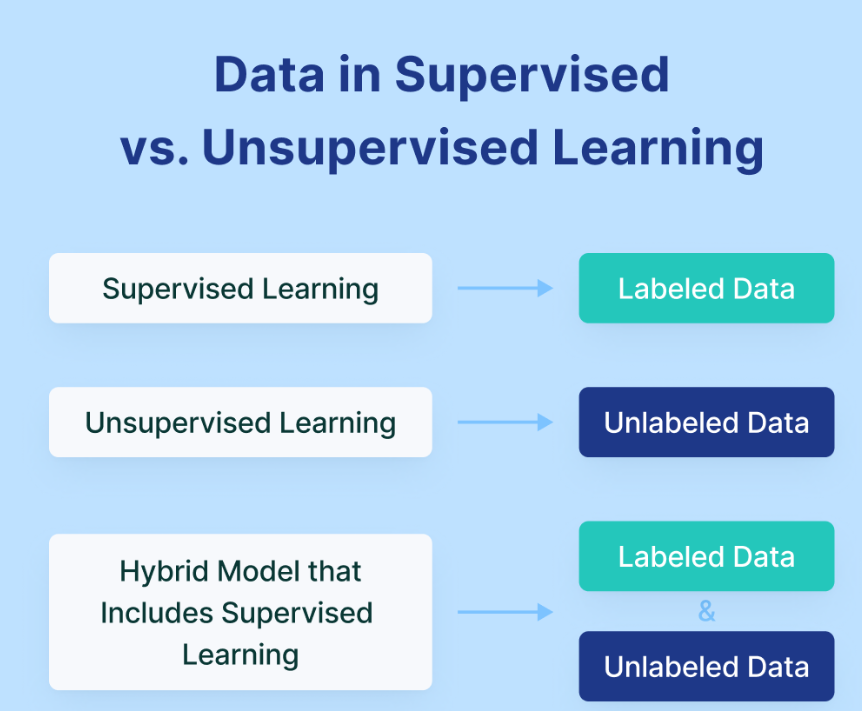
Reinforcement Learning
- Definition: Reinforcement learning involves an agent learning to make decisions by interacting with an environment. The agent learns from the consequences of its actions, aiming to maximize a reward signal. Â 1. github.com github.com
- Process: The agent explores the environment, taking actions and receiving rewards or penalties. Over time, the agent learns to choose actions that maximize the cumulative reward.
- Common Algorithms: Q-learning, Deep Q-Networks (DQN), Policy Gradient Methods, Actor-Critic Methods.
- Applications: Robotics, game playing, finance, healthcare, recommendation systems.
Comparison Table
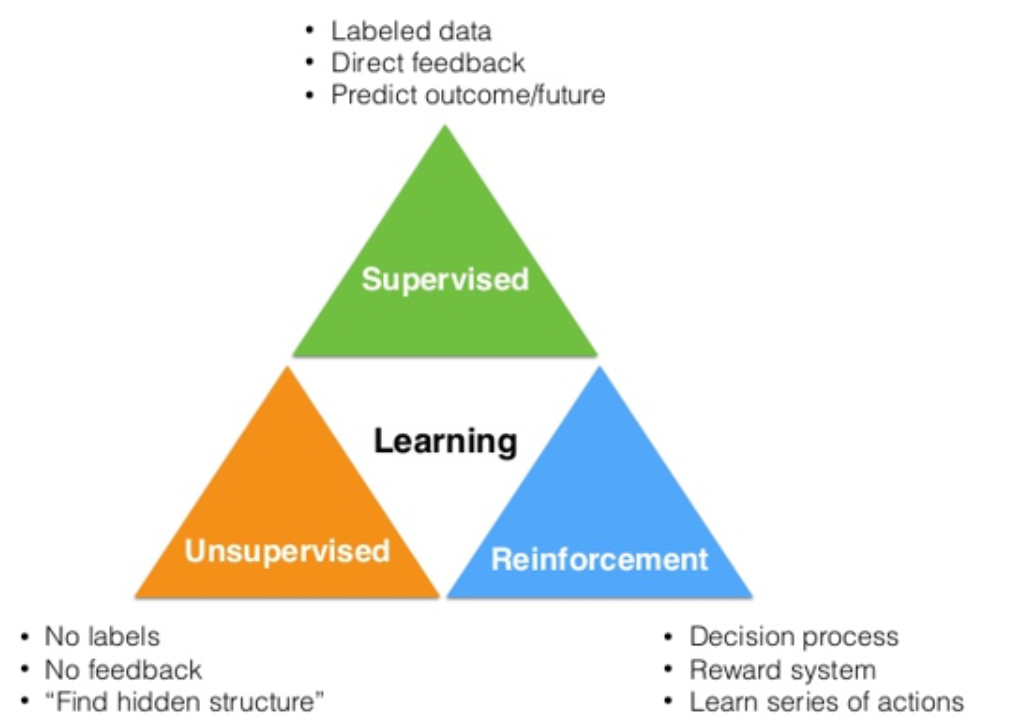
Feature | Supervised Learning | Unsupervised Learning | Reinforcement Learning |
---|---|---|---|
Data | Labeled | Unlabeled | No explicit labels |
Goal | Predict output based on input | Find patterns and structures | Learn optimal actions to maximize rewards |
Feedback | Teacher provides correct answers | No external feedback | Environment provides rewards or penalties |
Common Algorithms | Linear Regression, Logistic Regression, Decision Trees | K-means, Hierarchical Clustering, PCA | Q-learning, DQN, Policy Gradient |
Applications | Image recognition, spam filtering, fraud detection | Customer segmentation, image clustering, anomaly detection | Robotics, game playing, finance |
Choosing the Right Approach
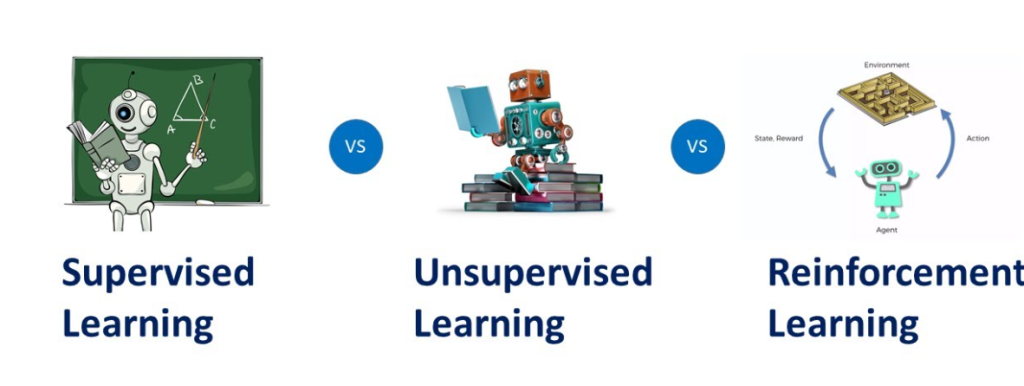
The choice of supervised, unsupervised, or reinforcement learning depends on the specific problem and the available data.
- Supervised learning is suitable when you have labeled data and a clear target variable to predict.
- Unsupervised learning is useful for exploring data, finding hidden patterns, and grouping similar data points.
- Reinforcement learning is applicable when dealing with sequential decision-making problems and when there’s a clear reward signal.
In many real-world scenarios, a combination of these techniques can be used to achieve better results. For example, unsupervised learning can be used to preprocess data before applying supervised learning models.
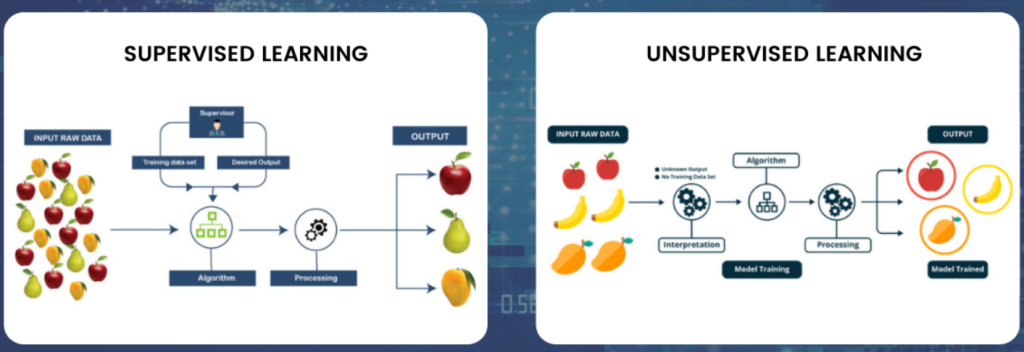