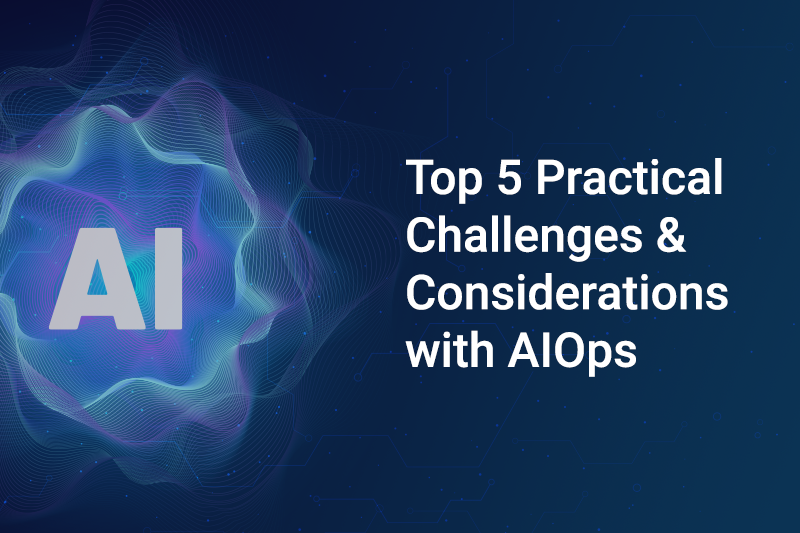
Artificial Intelligence for IT Operations, also known as AIOps, is a rapidly growing field that combines machine learning and big data analytics to automate and enhance IT operations. AIOps solutions aim to improve the efficiency and reliability of IT systems by identifying and resolving issues before they become critical.
While AIOps has the potential to revolutionize IT operations, it also faces several challenges that need to be addressed. In this article, we will explore the challenges for AIOps and how they can be overcome.
Challenge 1: Lack of Data Quality
One of the primary challenges for AIOps is the lack of data quality. AIOps solutions rely on large amounts of data to train machine learning algorithms and make accurate predictions. However, data quality is often poor, with missing, incomplete, or inaccurate data.
To overcome this challenge, organizations must focus on improving data quality by implementing data management strategies, such as data cleaning, normalization, and enrichment. They must also invest in data governance practices to ensure data accuracy and consistency.
Challenge 2: Complexity of IT Systems
IT systems are becoming increasingly complex, with multiple layers of infrastructure and applications. AIOps solutions must be able to handle this complexity and provide insights across the entire IT environment.
To address this challenge, AIOps solutions must be designed to integrate with various IT systems and provide a unified view of the entire IT environment. This requires a holistic approach to IT operations management and the ability to identify and correlate events across different systems.
Challenge 3: Lack of Expertise
Another challenge for AIOps is the lack of expertise in machine learning and big data analytics. AIOps solutions require skilled professionals who can design, implement, and maintain complex machine learning algorithms.
To overcome this challenge, organizations must invest in training and development programs to build a skilled workforce. They must also collaborate with external partners who can provide expertise in machine learning and big data analytics.
Challenge 4: Resistance to Change
Organizations may be resistant to change and reluctant to adopt new technologies, especially if they have already invested heavily in traditional IT operations management tools.
To address this challenge, AIOps vendors must provide clear and compelling business cases for their solutions. They must also demonstrate the value of AIOps by showcasing real-world use cases and success stories.
Challenge 5: Ethical Considerations
As AIOps solutions become more advanced, they may raise ethical concerns, such as data privacy and bias.
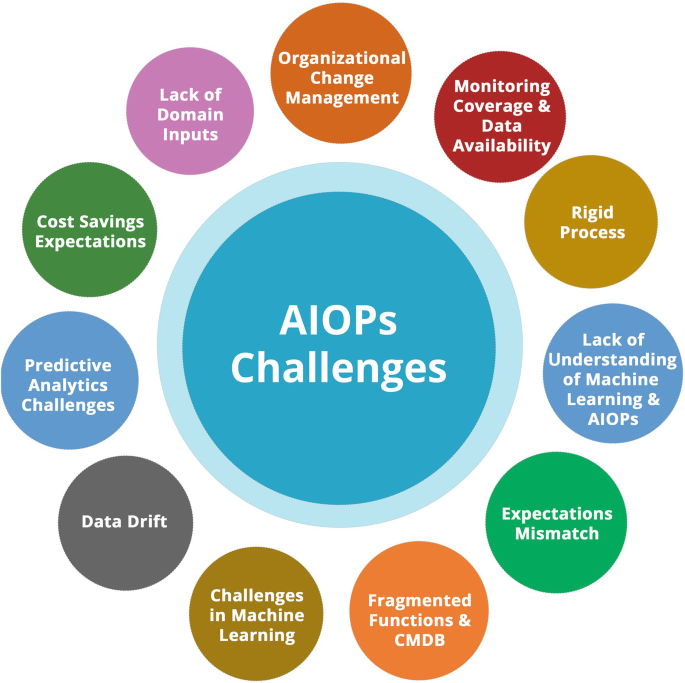
To address this challenge, organizations must adhere to ethical principles and best practices when designing and implementing AIOps solutions. They must also prioritize transparency and accountability to build trust with their customers.
In conclusion, AIOps has the potential to transform IT operations by automating and enhancing processes. However, it also faces several challenges, such as data quality, complexity of IT systems, lack of expertise, resistance to change, and ethical considerations. By addressing these challenges, organizations can unlock the full potential of AIOps and improve the efficiency and reliability of their IT systems.