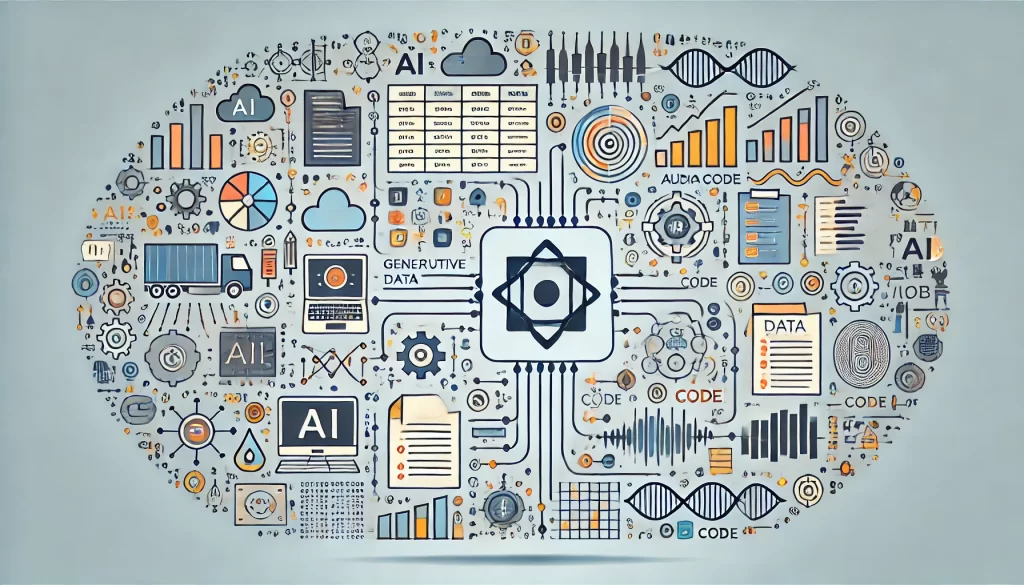
The development of generative AI has made tremendous strides in recent years, opening doors to creative, educational, and commercial applications. Behind the magic of generative AI lies an essential ingredient: datasets. From text and image generation to creating music and synthesizing complex data patterns, the quality and diversity of datasets used in training these models are crucial to their performance and capability. In this blog post, we’ll explore the types of datasets used to train generative AI models, their sources, and the significance of choosing the right data.
Types of Datasets for Generative AI
The type of data used depends largely on the model’s intended purpose—whether it’s generating realistic conversations, creating images, synthesizing voices, or producing video. Here’s an overview of the common types of datasets:
- Text Datasets: Text-based models are trained on vast language corpora, including books, articles, conversations, and online discussions. These datasets help AI understand linguistic nuances, idioms, and complex structures for generating coherent and contextually appropriate text.
- Image Datasets: Models focused on generating or interpreting images need extensive collections of labeled visuals to learn colors, shapes, textures, and object relationships. Many datasets pair images with descriptive text to give the model a richer understanding of visual context.
- Audio Datasets: Audio-based generative models rely on datasets of recorded speech, environmental sounds, or musical elements. These allow models to learn the unique patterns of human speech, background noises, and musical notes for generating realistic audio.
- Video Datasets: For video generation and interpretation, datasets often consist of clips annotated with actions, scenes, and context, enabling models to capture temporal dynamics and interactions.
- Multimodal Datasets: Some generative models, like CLIP and DALL-E, are designed to process multiple forms of data (e.g., images with captions or audio with transcriptions). Multimodal datasets provide multiple data types together, teaching models to correlate information across different formats.
Popular Datasets for Generative AI
Let’s explore some of the widely-used datasets across different data types, along with their primary applications.
A. Text Datasets
- Common Crawl: One of the largest publicly available datasets, Common Crawl includes billions of web pages, offering diverse content in multiple languages. It’s often used to provide models with a broad understanding of conversational and formal text.
- Wikipedia: A well-curated source of structured, factual content, Wikipedia offers models a reliable foundation for general knowledge. It’s commonly used for training models that require accuracy and a wide range of topics.
- BooksCorpus: This dataset is composed of text from over 11,000 books, providing longer, coherent text samples that help models learn narrative and structural patterns. It’s ideal for generative models focused on creative writing and storytelling.
- OpenWebText: This dataset was developed as an open-source alternative to OpenAI’s WebText. It includes high-quality web pages with diverse language and style, covering a range of topics, which helps language models in understanding varied writing conventions.
- News Datasets (e.g., RealNews): News datasets provide structured, timely information on current events, making them valuable for models that need to understand formal language and current topics.
B. Image Datasets
- ImageNet: With over 14 million labeled images across 20,000 categories, ImageNet has been instrumental in advancing computer vision tasks. It provides the basis for models trained to recognize or generate images of objects and scenes.
- COCO (Common Objects in Context): COCO contains 330,000+ images with detailed annotations, often with multiple objects in various scenes, enabling models to understand relationships and contextual placements.
- Flickr8k, Flickr30k, and MSCOCO Captioning: These datasets consist of images with descriptive captions, ideal for training on image-to-text tasks like caption generation and for understanding the relationship between visuals and language.
- LAION-5B: An open-source dataset with over 5 billion image-text pairs, LAION-5B is commonly used in training multimodal models like CLIP and DALL-E. Its large scale makes it valuable for understanding image-text relationships in a wide range of contexts.
- CelebA: This dataset of celebrity faces is frequently used for face-related generative tasks, like face synthesis, recognition, and enhancement.
C. Audio Datasets
- LibriSpeech: Based on audiobook recordings, LibriSpeech provides around 1,000 hours of transcribed English speech, which is valuable for training and testing speech recognition and generation models.
- VoxCeleb: This large-scale audio dataset consists of speech from thousands of individuals across demographics. It’s particularly useful for models that require an understanding of speaker-specific characteristics, such as voice generation and impersonation.
- UrbanSound8K: UrbanSound8K includes audio samples of common urban sounds, such as sirens, street noise, and more. This dataset is useful for training models that need to distinguish environmental sounds.
- Common Voice by Mozilla: A crowd-sourced voice dataset with a diverse range of languages, accents, and speaker demographics, Common Voice supports multilingual and accent-inclusive voice recognition models.
D. Video Datasets
- Kinetics: With hundreds of thousands of clips showing different human actions, Kinetics helps models learn motion patterns, making it ideal for action recognition tasks and movement understanding.
- YouTube-8M: YouTube-8M is a large video dataset with millions of video URLs labeled with content categories. It’s useful for models trained to recognize video content patterns, making it suitable for video recommendation systems and summarization models.
- AVA (Atomic Visual Actions): AVA is focused on short video clips labeled with atomic actions, helping models understand the basics of human interactions and movement.
E. Multimodal Datasets
- Visual Genome: Visual Genome offers images annotated with objects, attributes, and relationships, and is used to train models that require a nuanced understanding of image-text relationships.
- Conceptual Captions: This dataset is composed of images and descriptive captions gathered from the web, enabling text-to-image and image-to-text models to understand how text can describe visuals accurately.
- SQuAD (Stanford Question Answering Dataset): Though primarily a text dataset, SQuAD is valuable for training models on question-answering tasks, often in combination with visual data in multimodal applications.
- CLIP (Contrastive Language–Image Pretraining): Trained on 400 million image-text pairs, CLIP helps models understand complex visual and textual relationships, powering a range of applications from image classification to text-to-image generation.
Challenges and Considerations in Dataset Selection
Selecting the right dataset for training generative AI models isn’t as simple as just picking the largest dataset available. Here are some important considerations:
- Data Quality: High-quality, well-annotated data is essential to avoid noisy, inaccurate outputs. High-quality datasets improve the accuracy and coherence of generated content.
- Diversity: A diverse dataset helps the model generalize better, reducing the risk of overfitting to specific patterns. It also broadens the model’s understanding, making it more versatile across topics, cultures, and languages.
- Bias and Ethical Considerations: Many datasets reflect biases present in society or the data source. It’s important to curate and preprocess data carefully to minimize the perpetuation of harmful stereotypes and biases.
- Data Size: Although larger datasets can improve model performance, they also require significant computational power and storage resources. The dataset’s size should balance with the available computing infrastructure.
- Privacy: Some datasets, especially those containing user-generated or sensitive content, require careful handling to protect privacy. Data anonymization, consent, and ethical sourcing are crucial to comply with privacy standards.