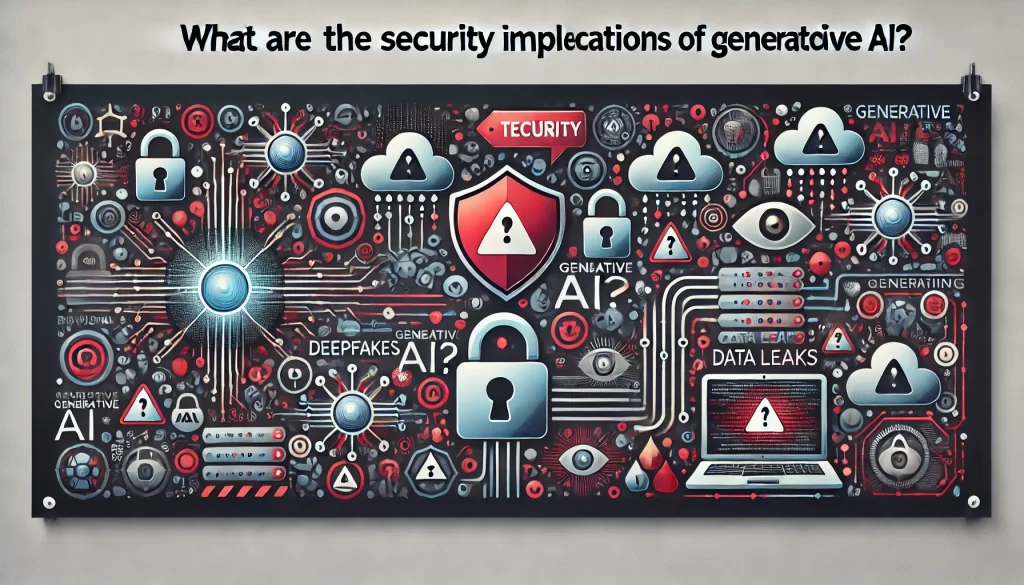
Generative AI is a groundbreaking technology with applications across industries, from content creation and healthcare to entertainment and education. However, its capacity to produce highly realistic content—including text, images, audio, and video—also introduces a range of security challenges. As generative AI models become more accessible and sophisticated, they bring both positive impacts and new security risks. In this blog, we’ll explore the security implications of generative AI, the potential threats it poses, and measures that can be taken to mitigate these risks.
Deepfakes and the Threat of Misinformation
One of the most widely recognized security concerns associated with generative AI is the rise of deepfakes—hyper-realistic videos, images, or audio created to impersonate individuals. While deepfake technology has legitimate uses, such as in entertainment or gaming, it can also be misused to spread misinformation or deceive audiences.
- Security Implications:
- Political Misinformation: Deepfakes can be used to create fake political speeches or statements, influence public opinion, cause civil unrest, or manipulate election outcomes.
- Corporate Espionage: Deepfake audio can impersonate corporate executives, leading to “CEO fraud” where attackers deceive employees into transferring money or disclosing sensitive information.
- Identity Theft and Blackmail: Deepfakes can impersonate individuals in compromising situations, leading to extortion or reputation damage.
- Solutions:
- Deepfake Detection Tools: AI models are now being trained to identify deepfakes by detecting inconsistencies in facial movements, lighting, or audio cues.
- Digital Watermarking: Embedding watermarks in authentic media content helps verify authenticity. Tools are being developed to add and detect these watermarks, marking content as genuine.
- Legal and Policy Measures: Some governments are implementing laws to penalize the malicious use of deepfakes and regulate the distribution of false information.
Example: A malicious actor creates a deepfake video of a prominent CEO making a damaging announcement. The video could cause panic among investors, leading to a drop in the company’s stock price and impacting its financial stability.
Phishing and Social Engineering Attacks
Generative AI can be used to automate and enhance phishing attacks by creating highly customized and convincing phishing messages. These messages can mimic the style, tone, and vocabulary of trusted sources, making it difficult for recipients to recognize them as fraudulent.
- Security Implications:
- Spear Phishing: Generative AI can gather information on a target from public data, creating personalized phishing emails that are harder to detect.
- Voice Phishing (Vishing): AI-generated voices can mimic real people, such as company executives, leading to phone-based scams or fraudulent calls.
- Malicious Chatbots: Attackers can deploy AI-powered chatbots to engage with users, collecting personal information or directing them to malicious sites.
- Solutions:
- Advanced Spam Filters: AI-driven filters can detect and block phishing emails, but they need to adapt to generative AI’s sophistication, focusing on context and subtle linguistic patterns.
- User Education and Awareness: Educating users about phishing tactics and encouraging skepticism about unsolicited messages helps reduce susceptibility.
- Two-Factor Authentication (2FA): Requiring 2FA for sensitive transactions adds a layer of security, making it harder for attackers to succeed with AI-driven social engineering.
Example: An AI-driven phishing email, appearing to come from a company’s HR department, could ask employees to update personal details on a fake portal, compromising sensitive information if employees aren’t vigilant.
Automated Malware Creation
Generative AI’s ability to write code can potentially be misused to create sophisticated malware or ransomware. This automation can generate complex code that’s harder for traditional security systems to detect and respond to.
- Security Implications:
- Polymorphic Malware: AI can help create malware that changes its code with each infection, making it difficult for antivirus software to recognize it by signature.
- Targeted Ransomware: AI can analyze network structures and user behaviors, creating ransomware that exploits specific weaknesses in a target’s systems.
- Stealthier Attacks: Generative AI can produce malicious scripts that mimic benign code, allowing them to bypass traditional defenses.
- Solutions:
- AI-Powered Threat Detection: Security systems using AI to detect unusual patterns in network behavior can identify and flag potential threats, even if they exhibit polymorphic characteristics.
- Behavioral Analysis: Rather than relying solely on code signatures, security systems can analyze behavior to identify unusual activities indicative of malware.
- Code Auditing Tools: Regular code audits and employing tools that identify AI-generated scripts can help prevent generative AI from being used to insert malicious code into systems.
Example: A polymorphic malware generated by AI could target healthcare institutions, encrypting patient records and demanding ransom without being easily identifiable by antivirus programs.
Intellectual Property Theft and Data Privacy
Generative AI models trained on vast datasets may inadvertently reproduce or expose sensitive information, intellectual property, or private data included in the training data. This can lead to breaches in data privacy and intellectual property theft.
- Security Implications:
- Data Leakage: Sensitive data, if present in the training set, might be unintentionally reproduced by generative AI models, leading to data breaches.
- Intellectual Property Infringement: Generative AI might produce outputs that closely resemble copyrighted works, raising concerns about intellectual property rights.
- Re-identification Risks: Even anonymized data can be used by AI models to identify individuals when combined with other datasets.
- Solutions:
- Federated Learning: This decentralized approach trains AI models without directly accessing raw data, reducing the risk of data exposure.
- Data Filtering and Sanitization: Before training, datasets should be carefully filtered to remove any identifiable information or proprietary data.
- Compliance with Privacy Laws: Ensuring compliance with GDPR, CCPA, and other data privacy regulations helps safeguard against unintentional data leakage.
Example: A generative AI model inadvertently recreates confidential business information included in its training data, leading to a data leak that exposes trade secrets.
Weaponization and Cyber Warfare
The power of generative AI can be exploited in cyber warfare, where AI models are used to generate disinformation, conduct large-scale cyber-attacks, or develop weapons with autonomous capabilities.
- Security Implications:
- Disinformation Campaigns: AI-generated propaganda or false information can influence public opinion, disrupt societies, and undermine political stability.
- Autonomous Weapons: Generative AI can be used to design and optimize weapon systems that operate autonomously, raising ethical and security concerns.
- AI-Powered Cyber Attacks: Generative AI can automate and scale cyber-attacks, targeting critical infrastructure like power grids, financial institutions, or government networks.
- Solutions:
- International Regulations: International agreements and treaties are necessary to limit the weaponization of AI and establish norms for its use.
- AI-Driven Defense Systems: Using AI to monitor for large-scale disinformation or cyber-attacks can help counteract AI-driven threats.
- Public Awareness: Educating the public about disinformation helps build resilience against AI-generated propaganda and fake news.
Example: In a cyber-warfare scenario, an attacker might use AI-generated deepfakes to create fake messages from government officials, leading to confusion and potential societal unrest.
Misuse in Financial Fraud
Generative AI can facilitate financial fraud by producing convincing synthetic identities, generating realistic fake documents, and automating complex scams. This increases the sophistication and scale of fraudulent activities.
- Security Implications:
- Synthetic Identity Fraud: AI can create synthetic identities that appear real, making it easier for criminals to commit fraud, open fake accounts, or apply for loans.
- Document Forgery: Generative AI models can generate realistic IDs, financial statements, and official documents, enabling identity theft or fraudulent transactions.
- Stock Market Manipulation: AI-generated news articles or social media posts can manipulate stock prices by spreading false information about companies.
- Solutions:
- AI-Based Identity Verification: Financial institutions can use AI-driven tools to detect fake identities or documents by examining subtle inconsistencies.
- Enhanced Document Authentication: Technologies like blockchain can authenticate digital documents, ensuring they haven’t been tampered with.
- Real-Time Monitoring: AI models can monitor financial markets for unusual patterns indicative of fraudulent activity, such as large-scale buying or selling triggered by misinformation.