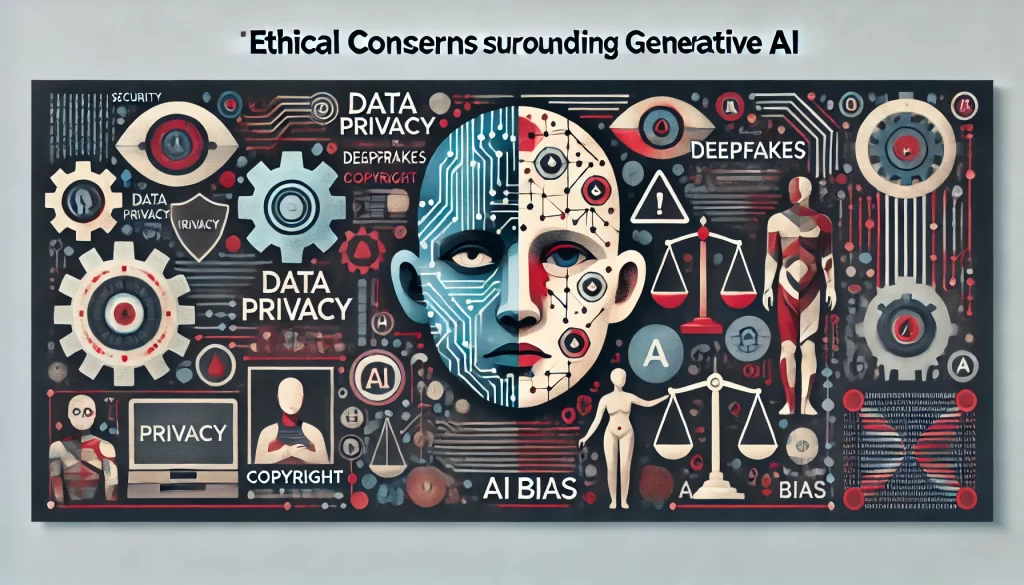
Generative AI has the potential to revolutionize various industries by producing realistic text, images, audio, and video. However, along with its many benefits, generative AI also raises significant ethical concerns. As these AI systems become more advanced, itโs essential to consider the ethical implications to prevent misuse and ensure responsible deployment. Below, we discuss the main ethical concerns surrounding generative AI and explore why they matter.
Bias and Fairness
Generative AI models learn from vast datasets, which often contain biases inherent in the data. These biases can be related to race, gender, culture, socioeconomic status, and more. When models learn from biased data, they risk reproducing or even amplifying these biases, leading to unfair or discriminatory outputs. This can have serious implications, especially in sensitive applications like hiring, law enforcement, and healthcare.
- Example: An AI-generated job description or recruitment tool might exhibit bias, inadvertently favoring certain demographic groups over others, leading to discrimination.
- Solution: Diverse and representative datasets, regular bias audits, and fairness-focused training methods can help mitigate this risk.
Misinformation and Deepfakes
Generative AI can produce highly realistic text, images, and videos, which can be used to create misinformation, fake news, and deepfake content. Deepfakesโvideos or images that realistically replace one personโs face with anotherโsโcan be used maliciously to spread false information, defame individuals, or manipulate public opinion. This has raised concerns about the potential for AI-generated content to mislead people on a large scale, affecting trust in media and information sources.
- Example: Deepfake videos could depict public figures saying or doing things they never did, potentially influencing public opinion or even elections.
- Solution: Watermarking AI-generated content, developing deepfake detection tools, and enforcing regulations to penalize malicious use are some ways to counteract this risk.
Intellectual Property and Ownership
Generative AI models are often trained on vast datasets that include copyrighted material, such as books, music, images, and videos. This raises questions about intellectual property rights and ownership. For instance, if a generative AI model produces artwork or music that resembles existing copyrighted works, who owns the output, and is it considered original?
- Example: An AI model trained on famous artistsโ works may produce new art that closely resembles copyrighted pieces, potentially infringing on intellectual property.
- Solution: Establishing clear guidelines on data usage, respecting copyright laws, and giving proper credit to original creators can help protect intellectual property rights.
Privacy and Data Security
Generative AI models can inadvertently reveal sensitive information if they are trained on personal or confidential data. For instance, language models trained on emails, chat logs, or other personal data could generate responses that include private information or confidential business details. This raises serious concerns about data privacy, especially in industries handling sensitive information.
- Example: A language model used in customer service could accidentally disclose personal information from previous interactions.
- Solution: Ensuring that data used for training is anonymized and that sensitive data is excluded can help preserve privacy. Implementing strict data governance policies is also essential.
Loss of Human Creativity and Jobs
Generative AI is capable of creating art, writing, music, and more, which raises concerns about the displacement of human creativity and jobs. As AI becomes more capable of producing content autonomously, thereโs a risk that it could replace human workers in creative fields, including graphic design, content creation, and music composition. This could result in economic challenges and a potential loss of human touch in creative works.
- Example: Companies might rely on AI to write articles or generate images, potentially reducing opportunities for human writers and artists.
- Solution: While AI can augment creative processes, preserving opportunities for human creators and recognizing the value of human input can help maintain a balance.
Accountability and Transparency
As generative AI becomes more autonomous, accountability for its outputs becomes a challenge. If an AI system generates harmful or biased content, itโs often unclear who should be held responsibleโthe developer, the user, or the AI itself. Additionally, many generative models operate as โblack boxes,โ meaning their decision-making processes are opaque, making it difficult to understand or explain how certain outputs were generated.
- Example: If an AI chatbot produces harmful or misleading information, it may be unclear whether the responsibility lies with the developer or the organization deploying it.
- Solution: Ensuring transparency in AI design, providing detailed documentation, and establishing accountability frameworks can clarify responsibilities for AI-generated content.
Environmental Impact
Training large generative AI models requires substantial computational resources, which consume significant energy and contribute to carbon emissions. As demand for AI models increases, so does the environmental footprint of training and running these systems. This raises concerns about sustainability and the long-term impact of AI on the environment.
- Example: Training a large language model can consume as much energy as several households do over an entire year, contributing to CO2 emissions.
- Solution: Adopting more energy-efficient algorithms, using renewable energy sources, and optimizing model architectures can help reduce the environmental impact of AI.
Emotional Manipulation and User Dependency
Generative AI can be designed to mimic human emotions and create empathetic responses, which, while useful, can also be manipulative. This is particularly concerning in applications involving vulnerable individuals, such as mental health chatbots, as people may develop emotional attachments to AI systems, leading to dependency or exploitation.
- Example: AI systems posing as friends or emotional support providers could manipulate usersโ emotions, leading to dependency on the AI or influencing decisions.
- Solution: Establishing ethical guidelines for empathetic AI and ensuring clear communication that the AI is not human can help manage user expectations and reduce dependency.