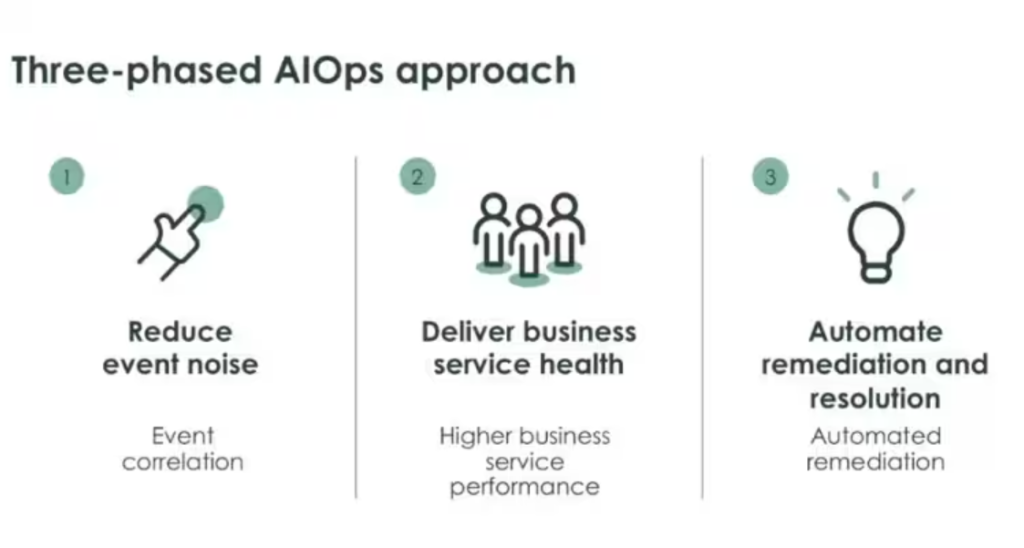
There are two main types of AIOps solutions:
- Domain-centric AIOps: These solutions are designed for a specific domain, such as cloud computing, networking, or security. They typically focus on a narrow set of problems and use domain-specific knowledge to improve the accuracy and efficiency of their solutions.
- Domain-agnostic AIOps: These solutions are designed to work across multiple domains. They typically use more general-purpose machine learning algorithms to identify patterns and anomalies in data.
Here are some of the most popular AIOps solutions:
- Datadog:Â Datadog is a monitoring platform that uses AI to help IT teams detect and resolve problems. It offers a wide range of features, including anomaly detection, root cause analysis, and incident response.
- New Relic:Â New Relic is another monitoring platform that uses AI to help IT teams improve their operations. It offers features for anomaly detection, performance monitoring, and application tracing.
- Splunk:Â Splunk is a data analytics platform that uses AI to help IT teams make sense of their data. It offers features for data collection, storage, and analysis.
- Moogsoft:Â Moogsoft is an AIOps platform that helps IT teams detect and respond to incidents. It offers features for anomaly detection, root cause analysis, and incident management.
- IBM Watson AIOps:Â IBM Watson AIOps is an AIOps platform that uses Watson AI to help IT teams improve their operations. It offers features for anomaly detection, root cause analysis, and incident response.
These are just a few of the many AIOps solutions available. The best solution for an organization will depend on its specific needs and requirements.When choosing an AIOps solution, organizations should consider the following factors:
- The size and complexity of the IT environment
- The specific problems that the organization wants to solve
- The budget
- The level of support that is required
Organizations should also be aware of the challenges of AIOps and take steps to address them. By doing so, they can make AIOps a valuable tool for improving IT operations.
Implementing AIOps (Artificial Intelligence for IT Operations) can be beneficial, but it also comes with several challenges that organizations need to address. Here are some common challenges associated with AIOps:
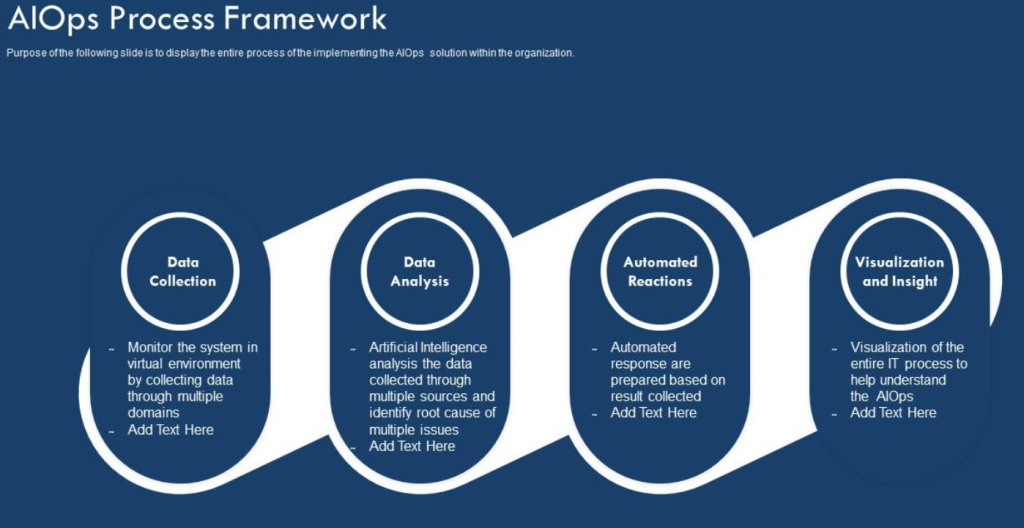
- Data Quality and Availability:
- AIOps relies heavily on accurate and high-quality data for analysis. Incomplete or inaccurate data can lead to unreliable insights and decisions.
- Data Integration:
- Organizations often have data spread across various systems, making it challenging to integrate and consolidate data for AIOps purposes.
- Complexity of IT Environments:
- Modern IT environments are complex, involving various technologies, platforms, and services. Capturing the entire landscape in an AIOps system can be difficult.
- Algorithm Selection and Tuning:
- Choosing the right algorithms and models for specific use cases is crucial for accurate predictions. However, this requires expertise in data science and machine learning.
- Model Bias:
- If training data contains bias, AIOps models can perpetuate these biases in their recommendations and decisions.
- False Positives and Negatives:
- AIOps systems can generate false alerts or miss important issues, affecting the trust that IT teams place in the system.
- Change Management:
- Implementing AIOps often involves changes in processes and workflows. Resistance to change and the need for training can slow down adoption.
- Skill Gap:
- Organizations might lack the required skills in data science and AI to effectively implement and manage AIOps systems.
- Privacy and Compliance:
- Handling sensitive data within AIOps systems raises concerns about data privacy and compliance with regulations like GDPR.
- Costs:
- Implementing AIOps involves costs for technology, training, and personnel. Organizations need to assess the return on investment.
- Monitoring and Maintenance:
- AIOps systems need constant monitoring and maintenance to ensure that algorithms remain accurate and relevant.
- Human-AI Collaboration:
- Organizations need to find the right balance between automated actions and human decision-making to avoid undue reliance on AI.
- Vendor Selection:
- Choosing the right AIOps vendor and solution can be challenging due to the variety of options available.
- Cultural Shift:
- AIOps requires a cultural shift towards data-driven decision-making and collaboration between IT and data science teams.
- Siloed Data and Teams:
- Lack of collaboration between different IT teams and data sources can hinder effective AIOps implementation.
- Interpretable AI:
- In critical IT environments, understanding how AI models arrive at decisions is essential. Some AI models can be difficult to interpret.