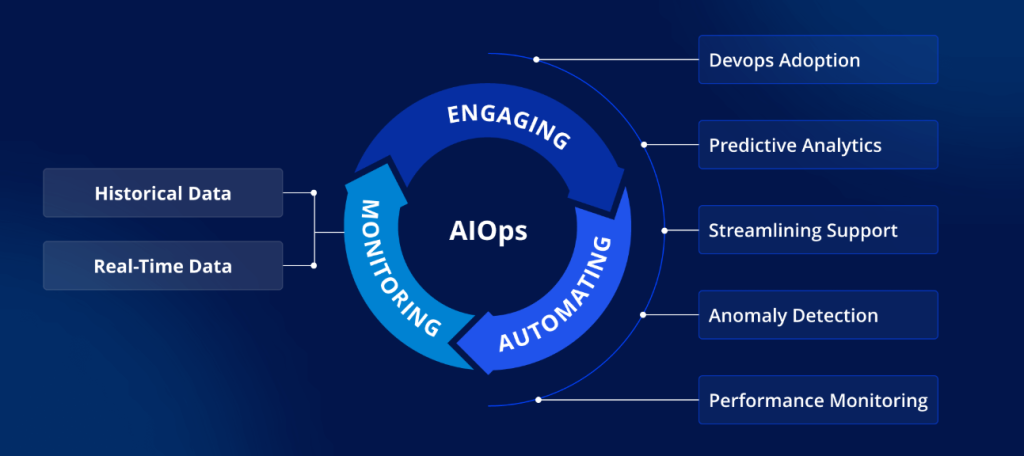
Introduction to AiOps Automation
The evolution of IT operations has reached a critical juncture where manual monitoring, rule-based alerts, and reactive troubleshooting are no longer sufficient to handle the complexity of modern IT environments. This is where AiOps AutomationโArtificial Intelligence for IT Operationsโsteps in to revolutionize how IT infrastructure, applications, and services are monitored, managed, and optimized.
AiOps leverages big data analytics, machine learning, and automation to offer real-time insights, automate incident responses, and ensure systems operate seamlessly with minimal human intervention. AiOps Automation helps organizations unlock:
- End-to-end observability across cloud, on-prem, and hybrid environments.
- Intelligent anomaly detection for proactive issue identification.
- Automated incident management and root cause analysis (RCA).
- Predictive capabilities to forecast issues before they impact business.
- Self-healing capabilities that trigger automated actions based on predefined policies.
With AiOps, IT teams transition from reactive firefighting to proactive, predictive, and preventative IT management, significantly improving service reliability and operational efficiency.
Key Features of AiOps Automation
Modern AiOps platforms offer a wide range of cutting-edge features that enable seamless IT operations across highly complex IT landscapes. These features ensure that both IT performance and customer experience are enhanced.
Real-time Data Aggregation and Analysis
- Collects data from logs, metrics, events, and traces across all infrastructure layers.
- Ingests data from monitoring tools, cloud platforms, network devices, and applications into a unified data lake.
- Applies machine learning models to correlate data and detect meaningful patterns in real time.
Intelligent Anomaly Detection and Predictive Alerts
- Identifies subtle performance anomalies that human operators might miss.
- Learns baseline behaviors and detects deviations in real-time.
- Generates predictive alerts, notifying teams of issues before they escalate into incidents.
Automated Incident Detection and Classification
- Classifies incoming alerts using AI models based on historical incident data.
- Prioritizes incidents based on business impact and criticality.
- Reduces alert fatigue by grouping related alerts into a single incident view.
Root Cause Analysis (RCA) with AI Assistance
- Analyzes historical events, log correlations, and configuration changes to pinpoint the exact root cause of problems.
- Reduces Mean Time to Resolution (MTTR) by surfacing actionable insights in seconds.
Automated Remediation and Self-Healing
- Triggers automated scripts or workflows to resolve known issues without human intervention.
- Supports self-healing infrastructure where common issues are automatically fixed.
- Offers suggested resolutions for complex problems requiring manual approval.
Benefits of Implementing AiOps Automation
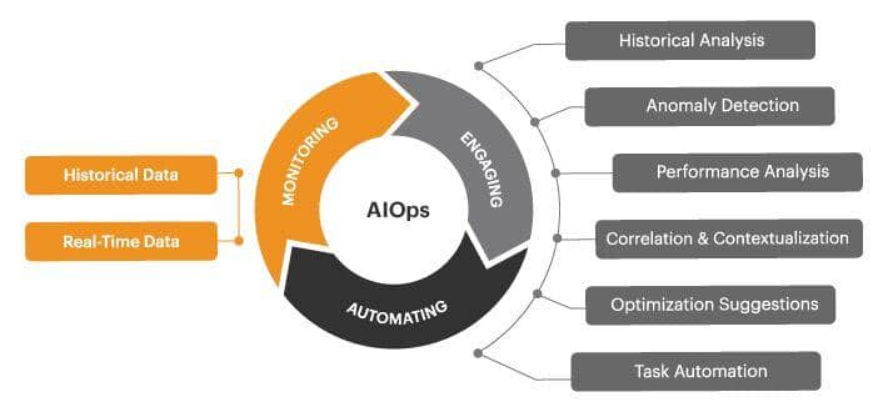
The adoption of AiOps isnโt just about technologyโit brings strategic business value by enhancing IT agility, reducing costs, and improving customer experiences. The core benefits include:
Enhanced Operational Efficiency
- Eliminates manual data correlation and incident triage tasks.
- Reduces the workload on IT teams by automating routine troubleshooting.
- Accelerates incident detection, classification, and response times.
Improved System Resilience and Uptime
- Detects performance degradation and anomalies early.
- Triggers automated fixes before end-users are impacted.
- Improves service reliability by enabling proactive maintenance and faster root cause identification.
Cost Optimization and Resource Efficiency
- Minimizes costs associated with downtime and incident resolution.
- Optimizes infrastructure usage through smart resource allocation.
- Reduces dependency on manual monitoring tools, saving licensing and operational costs.
Better Cross-team Collaboration
- Provides a single pane of glass view into IT health and performance.
- Ensures DevOps, IT Operations, and Business teams have access to the same data.
- Enhances coordination during critical incidents through AI-powered war rooms.
Enhanced Customer Experience
- Reduces outages and performance degradation, ensuring consistent user experiences.
- Enables faster service restoration, minimizing customer impact.
- Improves digital experience monitoring for proactive user satisfaction management.
Real-World Use Cases of AiOps Automation
AiOps has wide-ranging applications across IT operations, security, and business services. Below are practical use cases where AiOps is driving value:
Infrastructure Monitoring and Optimization
- Tracks health and performance of servers, VMs, containers, databases, and networks.
- Automatically scales resources up or down based on performance trends and forecasts.
- Identifies and corrects configuration drift across infrastructure components.
Application Performance Management (APM)
- Continuously monitors application response times, error rates, and user experiences.
- Detects code-level bottlenecks, enabling developers to optimize performance.
- Correlates application issues with underlying infrastructure problems.
Security Incident Detection and Response
- Detects unusual behaviors in network traffic, system logs, and application access patterns.
- Triggers automated response actions such as isolating compromised endpoints.
- Integrates with Security Information and Event Management (SIEM) tools for unified security management.
Automated Log Analysis and Event Correlation
- Correlates logs and events across disparate systems to uncover root causes of complex incidents.
- Groups related alerts into incident timelines, reducing noise and simplifying troubleshooting.
- Automatically identifies recurring issues, enabling permanent fixes.
Cost and Performance Optimization in Cloud Environments
- Monitors multi-cloud environments for cost and performance optimization.
- Identifies underutilized resources and suggests rightsizing options.
- Provides spend forecasts and optimization recommendations.
Challenges and Considerations When Adopting AiOps
Despite the transformative potential, successful AiOps implementation requires addressing key challenges. Organizations should plan for:
Data Integration Complexity
- Ingesting data from legacy systems, SaaS platforms, on-prem infrastructure, and multiple cloud providers.
- Ensuring data normalization and consistency across diverse data sources.
Model Accuracy and Continuous Learning
- Building accurate ML models requires high-quality historical data.
- Continuous model retraining is required to account for evolving IT environments.
- Balancing between false positives and missed alerts is critical.
Skills Gap and Cultural Change
- Upskilling existing IT teams in AI, data science, and automation technologies.
- Overcoming resistance to AI-driven decision-making in traditional IT organizations.
- Establishing cross-functional AiOps teams to drive adoption.
Privacy, Security, and Compliance
- Ensuring data privacy when ingesting logs, traces, and events containing sensitive information.
- Maintaining audit trails and compliance with industry regulations (GDPR, HIPAA, etc.).
- Implementing strong access controls and encryption.
Avoiding Vendor Lock-in
- Selecting AiOps platforms that support open standards and interoperability.
- Building architectures that can work with multi-cloud and hybrid infrastructures.
- Ensuring portability of AI models, automation scripts, and incident data.
The Future of AiOps: Emerging Trends
The AiOps landscape will continue evolving, with emerging trends enhancing its capabilities and expanding its role within IT and business ecosystems.
- Generative AI for AiOps: Leveraging GenAI for incident summaries, playbook generation, and RCA documentation.
- Digital Twins for IT Operations: Simulating infrastructure behavior to predict future issues.
- Proactive IT Service Management (ITSM): Shifting from reactive ticketing to automated incident prevention.
- Expanded Observability Platforms: Combining logs, metrics, traces, and user data into unified observability solutions.
- Edge and IoT Monitoring: Extending AiOps to edge devices and IoT infrastructure.