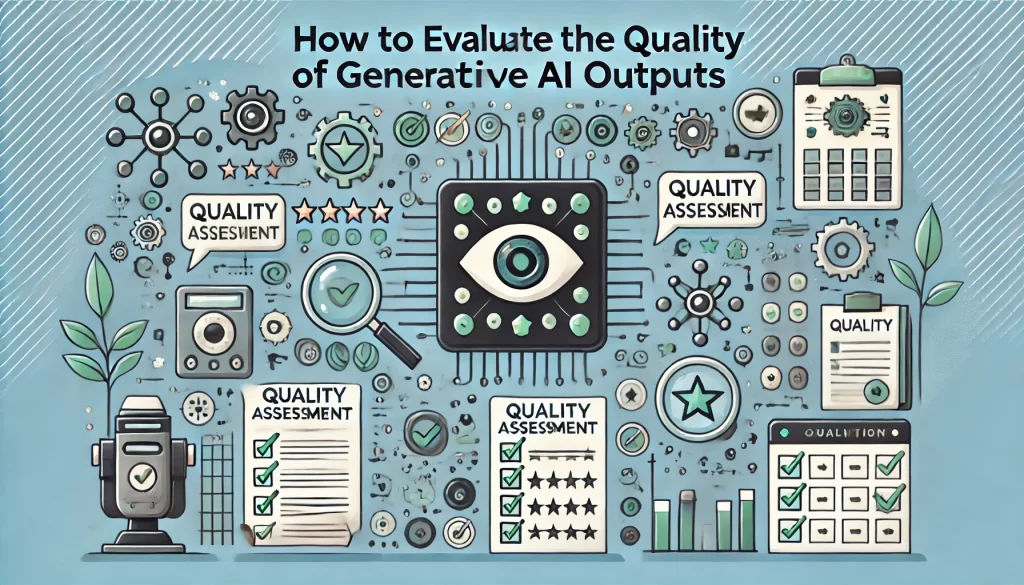
Generative AI has transformed how we produce content, allowing for the creation of realistic text, images, audio, and video. However, as impressive as these outputs can be, evaluating their quality is crucial to ensure accuracy, coherence, realism, and relevance. Unlike traditional AI, which often relies on clear right-or-wrong answers, generative AI produces new content with subjective qualities, making evaluation complex. This guide explores the essential metrics and techniques used to assess the quality of generative AI outputs across different media, helping developers, researchers, and businesses gauge the effectiveness of AI-generated content.
Coherence and Relevance
Coherence and relevance are fundamental when evaluating text-based generative AI, particularly in applications like content creation, customer service, or virtual assistants. Coherence refers to logical flow, ensuring that the generated content follows a clear, understandable structure. Relevance, on the other hand, determines if the content addresses the prompt or topic appropriately.
- Coherence: High coherence means that sentences logically connect without sudden shifts or irrelevant details. For instance, a coherent AI-generated story will have a beginning, middle, and end, with events following a logical sequence.
- Relevance: Relevance ensures the output aligns with the initial prompt or query. For instance, if asked to summarize a news article, the AI should not veer off into unrelated topics.
Metrics and Methods:
- BLEU Score (Bilingual Evaluation Understudy): Used to evaluate text coherence by measuring word sequence overlaps between AI-generated and reference texts. Although originally developed for translation, BLEU is widely used for text generation tasks.
- ROUGE Score (Recall-Oriented Understudy for Gisting Evaluation): Measures the overlap between AI output and reference summaries, particularly useful for tasks like summarization.
- Human Evaluation: Since coherence and relevance can be subjective, human evaluators provide valuable feedback, rating the output’s flow, clarity, and adherence to the prompt.
Example: In conversational AI, coherence ensures that the bot’s responses are logical, while relevance means the bot answers the user’s query accurately.
Fluency and Grammar
For any language-based generative AI, fluency and grammar are vital components that affect readability and professionalism. Fluency indicates how naturally the text reads, and grammar reflects the AI’s ability to follow language rules, including sentence structure, punctuation, and vocabulary.
- Fluency: Fluent AI-generated text is easy to read, with natural sentence flow and clear articulation of ideas.
- Grammar: Proper grammar ensures the text is accurate, free from errors, and appears professional. Poor grammar can reduce trust in AI, particularly in business or educational applications.
Metrics and Methods:
- Grammatical Error Rate (GER): Counts the number of grammatical errors in the generated output, with a lower error rate indicating higher quality.
- Automated Grammar Tools: Tools like Grammarly can highlight grammar issues, making them useful for automated evaluation of AI-generated text.
- Human Review: Human evaluators assess fluency and grammar, often providing insights into tone, word choice, and phrasing that automated tools might miss.
Example: In customer service emails, fluency ensures clear communication, while good grammar projects professionalism, helping to build customer trust.
Diversity and Creativity
In creative fields such as storytelling, art, or music, diversity and creativity are crucial. Diversity measures the variety in AI-generated outputs, while creativity assesses originality. These qualities prevent content from becoming repetitive or formulaic, ensuring that each output feels unique and innovative.
- Diversity: High diversity means the AI generates varied content for similar prompts, which is especially important in creative applications like storytelling or design.
- Creativity: Creative outputs offer novel ideas, patterns, or styles, showing the AI’s ability to push beyond predictable results.
Metrics and Methods:
- Self-BLEU Score: Measures diversity by comparing outputs generated from similar prompts, with a lower Self-BLEU score indicating greater diversity.
- Novelty Metrics: Assess how distinct the AI output is compared to training data, ensuring that generated content doesn’t overly mimic existing works.
- Human Evaluation: Human reviewers assess creativity, especially in open-ended tasks like art or storytelling, where originality and emotional impact are subjective.
Example: In content marketing, diversity ensures that an AI-powered social media tool doesn’t produce repetitive posts, while creativity enables the generation of engaging and unique campaigns.
Realism and Authenticity
For applications that generate images, audio, or video, realism and authenticity are paramount. Realism measures how accurately the AI replicates real-world characteristics, while authenticity assesses whether the output is believable within the intended context.
- Realism: In image and video generation, realism means outputs should look natural, with accurate lighting, textures, and proportions. In audio, realism is judged by the clarity and naturalness of speech or sound.
- Authenticity: Ensures the output aligns with context, avoiding uncanny or implausible elements. Authenticity is critical in fields like deepfakes or virtual simulations where the output needs to blend seamlessly with real elements.
Metrics and Methods:
- Inception Score (IS): Assesses the quality of generated images based on object recognition, with higher scores indicating clearer, more realistic outputs.
- Frechet Inception Distance (FID): Compares distributions of generated images and real images, with lower FID scores indicating outputs that resemble real images more closely.
- Mean Opinion Score (MOS): Commonly used in audio evaluation, where human raters score the naturalness of AI-generated speech.
Example: In virtual reality, realism ensures that generated environments feel lifelike, while authenticity helps create a believable immersive experience.
Consistency and Stability
Consistency measures whether an AI model generates outputs of similar quality across different inputs, while stability assesses the model’s ability to avoid erratic, unpredictable behavior.
- Consistency: Important in applications that require multiple outputs to fit together, such as generating different scenes in a game or sections in a story.
- Stability: Evaluate whether the AI reliably produces coherent and high-quality outputs without abrupt drops in quality, which is essential for user trust.
Metrics and Methods:
- Standard Deviation of Quality Scores: Measures consistency across outputs, with lower deviation indicating steady performance.
- Testing on Edge Cases: Evaluates how the AI handles complex or unusual inputs, revealing potential stability issues.
- Human Review: Human evaluators rate consistency and stability by examining outputs in different scenarios to identify inconsistencies.
Example: In educational content generation, consistency ensures that all explanations meet a standard of clarity, while stability ensures that AI responses remain helpful regardless of question difficulty.
Ethics and Bias Evaluation
Ethics and bias evaluation are crucial, particularly when generative AI is applied in sensitive areas like hiring, marketing, and media. Generative AI must avoid reinforcing harmful stereotypes or producing biased content.
- Bias Detection: This involves identifying and reducing any implicit biases that the model may have absorbed from its training data, such as stereotypes related to race, gender, or culture.
- Ethics Assessment: Ensures that AI-generated content adheres to ethical standards, particularly important in deepfake technology and content generation for public consumption.
Metrics and Methods:
- Bias Detection Algorithms: Analyze outputs for language or imagery that may contain biases, providing insights into where adjustments are needed.
- Crowdsourced Feedback: Uses input from diverse groups to assess inclusivity and fairness in AI outputs.
- Contextual Analysis: Examines how AI outputs fit within ethical guidelines, especially relevant for content like deepfakes or misinformation detection.
Example: In recruitment, bias evaluation ensures that AI-generated job descriptions or applicant summaries are free from gender or cultural biases.
User Satisfaction and Engagement
For applications like marketing, social media, and interactive platforms, user satisfaction and engagement are key indicators of quality. User satisfaction measures how well the AI’s output meets the audience’s needs, while engagement reflects the content’s ability to captivate users.
- User Satisfaction: Directly assesses how users feel about the AI’s output through feedback, surveys, or ratings.
- Engagement: Metrics like click-through rates, shares, and time spent on content provide insights into how engaging the AI-generated content is for its audience.
Metrics and Methods:
- User Surveys and Ratings: Collect direct feedback from users on the quality and relevance of AI-generated content.
- A/B Testing: Compares AI-generated outputs against alternative content to determine which performs better in user engagement metrics.
- Time-on-Task: Measures how much time users spend interacting with AI-generated content, providing insight into engagement levels.