
Introduction: The Cost of Downtime and the Need for Proactive IT
Downtime is one of the most expensive and disruptive challenges for modern businesses. Every minute of downtime can result in lost revenue, damaged customer trust, operational disruptions, and regulatory fines โ especially for businesses in sectors like finance, healthcare, and e-commerce. Traditionally, IT operations teams have relied on reactive approaches to monitor systems, troubleshoot issues, and restore services after problems arise.
However, with hybrid cloud environments, distributed microservices, containerized applications, and constantly shifting workloads, reactive IT operations are no longer sustainable. Businesses need to predict and prevent downtime before it affects end-users โ and this is exactly where AiOps (Artificial Intelligence for IT Operations) excels.
AiOps platforms use advanced machine learning, data analytics, and intelligent automation to not only detect and diagnose potential problems, but to forecast future issues and take preventive actions automatically.
Why Predicting and Preventing Downtime Matters
- Avoids revenue loss and customer churn.
- Preserves brand reputation and regulatory compliance.
- Minimizes operational disruptions across teams.
- Enables IT teams to focus on innovation, not firefighting.
- Delivers consistently high service availability.
Key Features of AiOps That Predict and Prevent Downtime
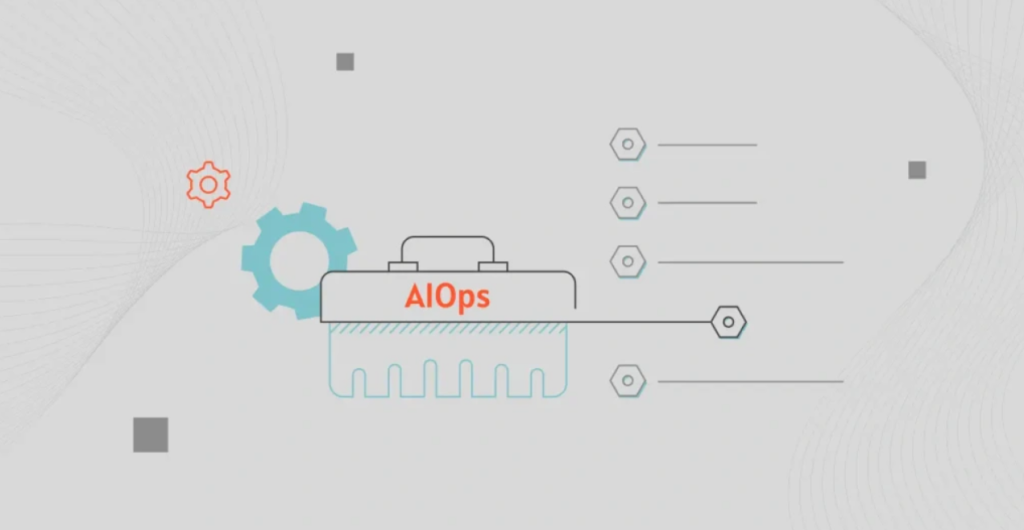
The ability of AiOps to predict and prevent downtime relies on a rich set of integrated features that go far beyond traditional monitoring tools. These capabilities provide early warnings, root cause analysis, and automatic remediation โ often before users notice issues.
Core Features of AiOps for Downtime Prevention
- Unified Observability and Cross-Domain Monitoring
- Aggregates data from servers, applications, networks, cloud platforms, containers, databases, and security tools.
- Correlates data across infrastructure, application, and business layers.
- Provides a real-time, end-to-end view of IT health.
- Machine Learning-Driven Pattern Recognition
- Learns what “normal” looks like across infrastructure components and services.
- Continuously updates baselines as workloads, user behavior, and deployments change.
- Detects deviations that might indicate emerging risks.
- Predictive Analytics and Forecasting
- Analyzes historical data to identify repeating failure patterns.
- Forecasts future failures based on current trends and environmental signals.
- Provides risk scores for services and systems.
- Real-Time Anomaly Detection and Correlation
- Identifies anomalies across logs, metrics, events, and traces.
- Correlates anomalies across dependent services to predict cascading failures.
- Prioritizes risks based on potential business impact.
- Automated Remediation and Prevention Playbooks
- Triggers pre-defined remediation workflows to prevent predicted downtime.
- Automates preventive actions like scaling, configuration tuning, or failover.
- Continuously refines playbooks based on past outcomes.
How AiOps Predicts Downtime Before It Happens
AiOps platforms don’t just react to problems; they forecast them using real-time analysis and historical learning. This ability to predict downtime in advance gives IT teams a critical window to respond before users are impacted.
The Process of Predicting Downtime with AiOps
- Data Collection Across All Layers
- Collects data from servers, cloud platforms, microservices, network devices, databases, and applications.
- Normalizes data for cross-domain correlation and machine learning analysis.
- Enriches operational data with configuration changes, code deployments, and user activity patterns.
- Learning Baselines and Detecting Early Deviations
- Learns normal behavior across infrastructure and services.
- Identifies deviations before they cross static thresholds.
- Tracks performance trends to spot slow-building risks.
- Correlation Across Events, Metrics, and Logs
- Links seemingly unrelated events across infrastructure and applications.
- Identifies multi-service anomalies that could lead to cascading failures.
- Builds a real-time risk map across the IT environment.
- Risk Scoring and Incident Prediction
- Assigns risk scores to systems and services based on:
- Past incidents and recurring patterns.
- Configuration drift and technical debt.
- Current performance degradation trends.
- Assigns risk scores to systems and services based on:
- Early Warning Alerts and Recommendations
- Provides proactive alerts with predicted timelines.
- Suggests corrective actions before issues escalate.
- Integrates with ITSM tools to automatically generate preventive tickets.
How AiOps Prevents Downtime with Intelligent Automation
The second half of the predict and prevent formula comes from intelligent automation โ the ability for AiOps to not only detect and predict issues, but to trigger automated actions to fix them or prevent them entirely.
The Process of Preventing Downtime with AiOps
- Automated Early Remediation
- Executes predefined self-healing workflows for recurring problems.
- Applies configurations adjustments, cache flushing, or service restarts automatically.
- Escalates only when manual intervention is required.
- Scaling and Resource Optimization
- Proactively scales resources when capacity limits are predicted.
- Dynamically rebalances workloads to avoid resource contention.
- Identifies and removes unused or redundant infrastructure.
- Change Impact Prediction and Validation
- Predicts how upcoming changes (code releases, config changes) could affect system health.
- Automatically tests changes in sandbox environments before deployment.
- Recommends rollback if predicted risks exceed thresholds.
- Cross-Service Dependency Risk Reduction
- Monitors upstream/downstream dependencies.
- Automatically applies preventive actions to related services if one component is at risk.
- Ensures system-wide resilience, not just isolated fixes.
- Continuous Learning and Playbook Enhancement
- Learns from every successful or failed automated action.
- Continuously refines self-healing playbooks.
- Builds a dynamic knowledge base to improve future prevention accuracy.
Benefits of Predicting and Preventing Downtime with AiOps
Predictive and preventive capabilities within AiOps deliver far-reaching benefits for both IT operations teams and business stakeholders.
Key Benefits for IT Operations and Business Teams
- Minimizes Customer-Impacting Outages
- Fixes issues before they become critical incidents.
- Prevents service disruptions across digital services, APIs, and backend systems.
- Ensures continuous customer experience with minimal interruption.
- Reduces Operational Costs
- Lowers the cost of incident response and manual troubleshooting.
- Reduces unplanned overtime and emergency staffing.
- Optimizes infrastructure spend by matching capacity to real demand.
- Accelerates Digital Transformation
- Frees IT teams to focus on innovation rather than constant firefighting.
- Supports more aggressive release schedules with lower risk.
- Improves confidence in cloud migrations and new technology adoption.
- Improves IT and Business Alignment
- Links system health to business impact metrics.
- Provides data-backed risk assessments to business leaders.
- Helps align IT investment decisions with uptime goals.
- Enhances Compliance and Reporting
- Documents all predicted risks, preventive actions, and outcomes.
- Provides audit trails for compliance and post-incident reviews.
- Strengthens risk management for regulated industries.
AiOps Is the Future of Proactive IT Management
In the age of digital-first business models, downtime is no longer acceptable. Predicting and preventing downtime with AiOps is no longer a luxury โ itโs a competitive advantage.
By combining predictive analytics, machine learning, intelligent automation, and real-time observability, AiOps transforms IT operations from reactive to proactive, ensuring:
- Higher uptime and reliability.
- Lower operational costs.
- Stronger IT-business alignment.
- Continuous service innovation.