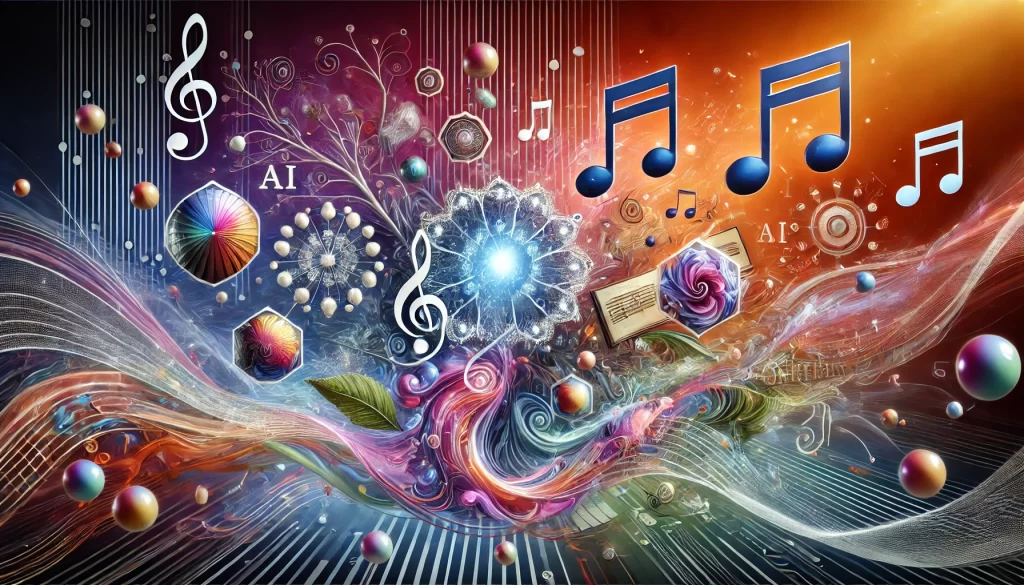
Generative AI is often described as a groundbreaking technology capable of creating new and original content, from images and music to text and videos. But what does it mean for AI to create “new” and “original” content? And can AI truly be innovative, or is it simply recombining patterns it has learned from existing data? In this blog, we’ll explore the extent to which generative AI can produce original content, examining its creative potential, the limitations it faces, and the ethical implications that arise.
What Does “Original Content” Mean in the Context of AI?
To understand whether AI can create original content, it’s essential to define what we mean by “original.” In human creativity, originality implies something novel, unique, and previously unseen. It often involves recombining existing ideas in fresh ways, introducing elements of innovation, or solving problems with new approaches.
In the case of generative AI, originality can be considered on two levels:
- Novel Combinations: AI can generate content that is unique in the sense that it has not appeared in its training data. By combining learned patterns, it can create novel outputs that resemble real-world content but are not direct replicas.
- True Creativity: Human creativity often involves intuition, emotions, and a deep understanding of context—qualities that AI lacks. Therefore, while AI can simulate aspects of originality, it doesn’t possess the same conscious intent or subjective experience that humans associate with creativity.
Example: A generative AI model trained on thousands of landscape paintings might produce a unique, unseen image by combining elements from different styles. While technically original, the image might lack the conscious intent or artistic message that a human artist would imbue in their work.
How Generative AI Creates New Content: An Overview of Model Techniques
Generative AI relies on sophisticated algorithms to produce new content. These algorithms have been fine-tuned to learn from large datasets and generate outputs that resemble, but are not identical to, the original data.
- Generative Adversarial Networks (GANs): GANs consist of two neural networks—a generator and a discriminator—that work together to create realistic outputs. The generator creates new content, while the discriminator evaluates it. Over time, the generator becomes skilled at producing images or videos that look realistic to human observers.
- Variational Autoencoders (VAEs): VAEs encode data into a compressed form, or “latent space,” and then decode it to produce new variations. VAEs are especially useful for generating content with specific attributes, such as images that share certain features with the training data but are structurally unique.
- Transformers and Large Language Models: Models like GPT-3 and ChatGPT, which are based on transformers, generate text by predicting and arranging words based on patterns learned from vast amounts of language data. These models can produce unique passages of text, answer questions, and even create narratives that are coherent and contextually relevant.
Example: Using GANs, AI can generate images of faces that are realistic but do not belong to any real person. The output is new in that it combines features from many faces in the training data to create something unique.
Can Generative AI Be Considered Creative? Exploring the Concept of Creativity in AI
Creativity is often seen as a uniquely human trait, involving imagination, intuition, and the ability to think abstractly. While generative AI can mimic these characteristics to a degree, it operates fundamentally differently from human creativity.
- Pattern Recognition and Replication: Generative AI learns patterns from its training data and recombines them in novel ways. It is not “aware” of what it is creating; it simply follows mathematical rules and probabilities to generate outputs that align with what it has learned.
- Limits of Understanding and Intent: AI does not understand or interpret the meaning of its creations, nor does it have any conscious intent. While it may produce text that sounds poetic or images that look aesthetically pleasing, it lacks subjective experience or intent to convey a message.
- Emergent Creativity: While AI may not be creative in the same sense as humans, it can produce outputs that surprise even its developers. This is often due to emergent patterns in complex models, where the AI generates combinations that were not explicitly programmed.
Example: GPT-3 can generate a story with plot twists and character development, which can feel “creative” to readers. However, the model does not “intend” to create suspense or drama—it’s simply generating text based on patterns learned from millions of narratives.
Applications of Generative AI in Creating New Content
Generative AI is widely used in industries that benefit from creative outputs, where new and unique content is valuable. Here are some of the most prominent applications:
- Art and Design: AI models like DALL-E and Midjourney can generate artwork based on text prompts, enabling artists to explore novel ideas and styles. These tools have become popular for producing unique concept art, posters, and illustrations.
- Writing and Storytelling: Language models like GPT-3 can write stories, poems, and articles that are coherent and contextually relevant. This has applications in content creation, journalism, and even literature, where writers use AI to brainstorm or generate first drafts.
- Music Composition: Generative AI can create music by learning patterns in rhythm, melody, and harmony. Tools like OpenAI’s MuseNet can compose songs in various genres, providing musicians with fresh ideas and inspiration.
- Product Design and Prototyping: AI can generate innovative designs for products, including furniture, vehicles, and fashion. By training in a variety of design styles, AI can propose new designs that blend functionality and aesthetics.
Example: In marketing, generative AI can create unique ad copy, social media posts, or even video content tailored to specific audiences, enabling brands to reach their customers with original, relevant material.
Challenges and Limitations: Can AI Be “Original”?
Despite its ability to produce unique outputs, generative AI has limitations when it comes to true originality. These challenges underscore the differences between AI-generated content and human creativity.
- Dependence on Training Data: AI’s outputs are heavily influenced by its training data. While it can recombine elements in novel ways, it cannot create concepts or styles that it hasn’t learned from the data.
- Risk of Repetition or Mimicry: Some generative models have been known to inadvertently replicate parts of their training data, especially when trained on smaller datasets. This raises questions about the originality of AI’s creations and potential copyright concerns.
- Lack of Intent and Interpretation: AI-generated content lacks the deeper layers of meaning or intent that often characterize human creativity. For example, a human artist may create a piece that reflects their personal experiences or emotions, while AI lacks this subjective context.
Example: A GAN trained in Impressionist art may produce an image that resembles Monet’s style but lacks the historical, cultural, and personal influences that shaped Monet’s work, making it an imitation rather than a genuine creation.
Ethical and Legal Considerations of AI-Generated Content
The use of generative AI to create new content raises several ethical and legal issues, particularly around originality and ownership.
- Intellectual Property and Copyright: If an AI model generates content similar to its training data, it could infringe on copyright laws, especially if that data includes copyrighted works. Determining who owns AI-generated content is complex, as traditional copyright law assumes a human creator.
- Plagiarism and Authenticity: AI-generated content can blur the line between inspiration and plagiarism, especially when models unintentionally replicate specific elements of their training data. This issue is particularly relevant in fields like art, music, and writing.
- Accountability for Misuse: Generative AI can produce misleading or harmful content, including deepfakes, fake news, or synthetic identities. Developers and users must consider the ethical implications and take steps to prevent misuse.
Example: A text generation model trained on news articles could produce content that appears to be credible but contains inaccuracies. If misused, this content could spread misinformation, raising questions about accountability and ethical responsibility.
Can Generative AI Foster Human Creativity?
While generative AI may not be creative in the human sense, it can still serve as a valuable tool for inspiring and enhancing human creativity.
- Creative Collaboration: Artists, writers, and musicians can use AI as a collaborator to generate new ideas, explore unfamiliar styles, or overcome creative blocks. AI can produce rough drafts or prototypes that humans can refine and personalize.
- Idea Generation: Generative AI provides a limitless source of new ideas, offering fresh perspectives that may not have occurred to the human creator. For example, AI-generated art or music can inspire artists to experiment with new techniques or styles.
- Augmenting Productivity: In fields like content marketing or product design, generative AI can quickly generate multiple iterations, allowing creators to focus on fine-tuning and optimizing ideas.
Example: A graphic designer might use DALL-E to generate initial design ideas, using the AI-generated images as inspiration before creating a polished, final piece that incorporates their style and vision.