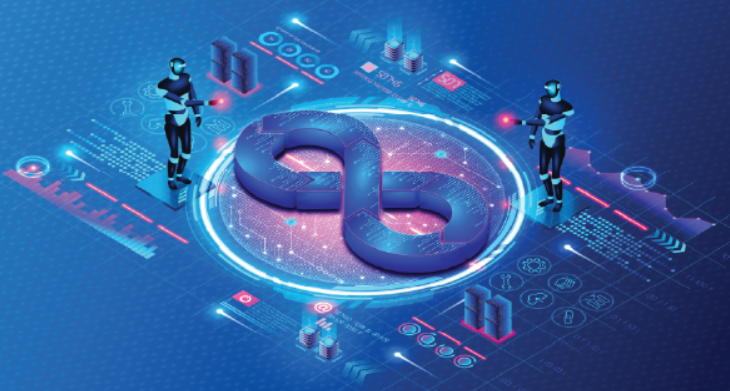
Introduction
In the ever-evolving landscape of IT management, the complexity of modern infrastructures has outgrown traditional monitoring methods. With the rise of cloud computing, microservices, and big data, IT systems have become increasingly intricate, requiring smarter solutions for monitoring and management. This is where AIOps (Artificial Intelligence for IT Operations) comes in, leveraging machine learning and data analytics to automate, predict, and optimize IT operations.
In this post, we will explore the differences between AIOps and traditional IT monitoring, discussing why AI is becoming the future of IT operations and how it addresses the shortcomings of traditional methods. We will highlight the major features of AIOps and show why it is transforming IT management.
1. What is Traditional IT Monitoring?
Understanding Traditional IT Monitoring
Traditional IT monitoring systems are built on manual processes and predefined thresholds for alerting. They rely heavily on rule-based systems and human intervention to monitor infrastructure, detect anomalies, and troubleshoot issues. These tools focus on collecting data from various systems, including servers, networks, and databases, and generating alerts when certain thresholds are breached.
Key Features of Traditional IT Monitoring:
- Rule-based Alerts: Relies on predefined thresholds to trigger alerts when certain conditions are met.
- Manual Configuration: IT teams manually configure rules and set up monitoring for different systems.
- Data Aggregation: Collects data from different sources but often lacks deep correlation between them.
- Human-Centric Troubleshooting: Requires manual intervention for issue diagnosis and resolution.
- Static Monitoring: Limited ability to adapt to changing environments and dynamic systems.
Traditional IT monitoring is often limited by the complexity and volume of modern IT environments. It struggles to keep up with the speed and scale of todayโs operations, leading to slower response times and higher chances of missing critical issues.
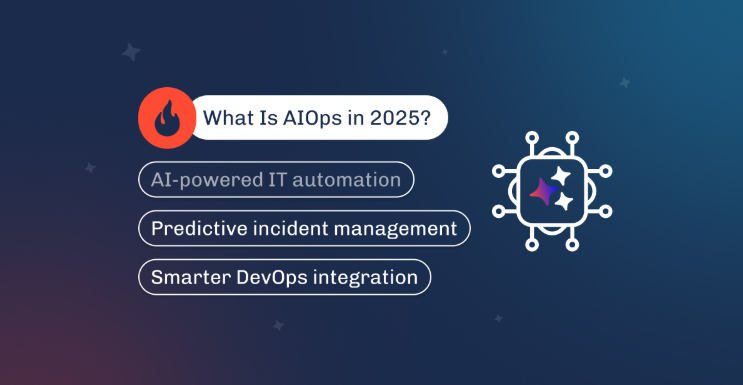
2. What is AIOps?
Understanding AIOps in IT Operations
AIOps is a new paradigm in IT operations that integrates artificial intelligence, machine learning, and advanced analytics to automate and optimize monitoring, incident management, and decision-making. Unlike traditional methods, AIOps can handle vast amounts of data in real-time, detect anomalies, predict potential issues, and even automatically resolve incidents without human intervention.
Key Features of AIOps:
- AI-Powered Anomaly Detection: Uses machine learning to automatically detect unusual patterns in data and predict potential failures.
- Event Correlation: Correlates events from various sources to identify the root cause of issues.
- Predictive Analytics: Leverages historical data and machine learning to predict and prevent incidents before they happen.
- Automated Incident Management: Automatically categorizes, prioritizes, and resolves incidents without requiring human intervention.
- Real-Time Data Processing: Processes large volumes of data in real-time for immediate insights and actions.
AIOps leverages AI and data science to continuously learn from operational data, improving its ability to detect, analyze, and resolve incidents automatically. This makes it an incredibly powerful tool for modern IT environments.
3. Key Differences Between AIOps and Traditional IT Monitoring
How AIOps and Traditional Monitoring Differ
While both AIOps and traditional IT monitoring aim to ensure the health and reliability of IT systems, they do so in vastly different ways. Below are the key differences between AIOps and traditional IT monitoring.
Key Differences:
- Data Processing:
- Traditional IT Monitoring: Often handles smaller datasets and processes them manually.
- AIOps: Processes massive datasets from multiple sources in real-time using AI algorithms.
- Incident Detection:
- Traditional IT Monitoring: Relies on predefined rules and static thresholds to detect issues, which may miss dynamic or unknown problems.
- AIOps: Uses machine learning to detect anomalies and uncover hidden patterns, providing more accurate and timely incident detection.
- Event Correlation:
- Traditional IT Monitoring: Struggles to correlate events across different systems, leading to fragmented insights.
- AIOps: Automatically correlates events across multiple platforms, improving root cause analysis and reducing incident resolution time.
- Automation:
- Traditional IT Monitoring: Requires manual intervention for incident response, troubleshooting, and system adjustments.
- AIOps: Automates the incident management process, triggering workflows to resolve incidents without human involvement.
- Scalability:
- Traditional IT Monitoring: Works well for smaller environments but struggles to scale in cloud-native or hybrid systems.
- AIOps: Built to scale, handling complex, dynamic environments, including cloud, microservices, and containers.
In essence, AIOps provides a smarter, more efficient, and automated approach to managing IT operations compared to traditional monitoring systems.
4. Why AIOps is the Future of IT Monitoring
The Rise of AI in IT Operations
With the complexity of modern IT environments and the increasing volume of data generated daily, traditional monitoring methods are becoming less effective. AIOps is the future because it brings automation, predictive capabilities, and smarter decision-making to IT operations.
Why AIOps is the Future:
- Real-Time Insights: AIOps provides real-time visibility and faster decision-making, ensuring that teams can respond quickly to incidents.
- Predictive Capabilities: With machine learning, AIOps predicts potential failures, minimizing downtime and improving system reliability.
- Automation: AIOps automates repetitive tasks like alerting, incident categorization, and remediation, freeing up valuable resources for more strategic activities.
- Improved Efficiency: By reducing the manual effort involved in incident response and monitoring, AIOps enhances operational efficiency.
- Adaptability: AIOps can evolve and adapt based on the continuous stream of operational data, making it future-proof for increasingly complex IT environments.
As organizations shift to cloud-native environments and embrace microservices, AIOps will become a critical component for managing dynamic and scalable infrastructures. Its ability to predict, automate, and optimize operations will make it indispensable for the future of IT management.
5. Real-World Use Cases of AIOps vs. Traditional IT Monitoring
AIOps in Action: Use Cases and Benefits
To truly understand the impact of AIOps, letโs explore how it is being used in real-world IT environments, and how it compares to traditional IT monitoring in practice.
Examples of AIOps in Action:
- E-Commerce Websites: AIOps tools help e-commerce platforms automate monitoring and predict traffic spikes, ensuring the systems are always ready to handle high demand.
- Cloud Providers: Cloud platforms use AIOps to automate infrastructure scaling and predict server failures, ensuring high availability and continuous uptime.
- Financial Institutions: Financial institutions leverage AIOps to monitor transaction systems, detecting fraudulent activity or performance issues in real-time, significantly reducing response times.
Traditional IT Monitoring Use Cases:
- Legacy Systems: Organizations with older, on-premises infrastructure rely on traditional monitoring tools to manage server health and network traffic, though these tools often require manual intervention.
- SMBs: Smaller businesses or those with less complex environments may still benefit from rule-based monitoring, although they face challenges in scaling as their operations grow.
While traditional monitoring still has its place in simpler IT environments, AIOps provides the speed, accuracy, and automation required for modern infrastructures.
6. The Road Ahead: How AIOps Will Evolve
The Future of AIOps in IT Monitoring
As AI and machine learning continue to advance, the role of AIOps in IT operations will only become more central. Here are a few ways AIOps is expected to evolve:
Future Trends:
- Deeper Integration with DevOps: AIOps will integrate more deeply with DevOps pipelines, automating CI/CD processes and improving release management.
- AI-Driven Self-Healing Systems: The future of AIOps will include self-healing systems that automatically resolve incidents with minimal human intervention.
- Expanded Use of Predictive Analytics: AIOps will further refine its predictive capabilities, enabling advanced forecasting for IT performance and resource management.
- Seamless Multi-Cloud Operations: AIOps will support increasingly complex hybrid and multi-cloud environments, helping organizations seamlessly manage IT resources across multiple platforms.
With the rise of edge computing, IoT, and 5G, AIOps will continue to evolve to manage next-gen IT infrastructures, ensuring speed, reliability, and security.
Conclusion
As IT environments grow in complexity and scale, traditional monitoring methods are struggling to keep up. AIOps, with its ability to predict, automate, and optimize IT operations, is rapidly becoming the future of IT monitoring. Its ability to detect issues in real-time, provide actionable insights, and automate incident response makes it an invaluable tool for modern IT teams.
While traditional IT monitoring may still be useful for smaller environments, AIOps is clearly the future for organizations that need to scale, adapt, and stay ahead of potential disruptions in their IT operations.