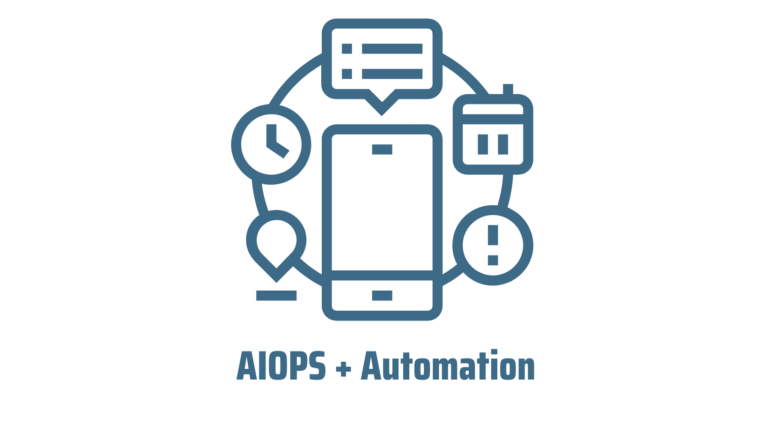
Introduction to AiOps Automation
In today’s rapidly evolving IT environments, the sheer scale, complexity, and speed of digital operations demand solutions that go beyond manual monitoring and management. The combination of hybrid cloud infrastructures, microservices architectures, containerized environments, and distributed applications has made it nearly impossible for human IT teams to manage operations efficiently without the risk of human errors.
This is where AiOps Automation steps in, acting as the next evolutionary leap in IT operations. AiOps (Artificial Intelligence for IT Operations) combines machine learning, data analytics, and automation to streamline IT operations, accelerate incident detection and resolution, and eliminate human errors from day-to-day processes.
How AiOps Automation Saves Time and Reduces Errors
- Automates repetitive operational tasks to eliminate manual intervention.
- Continuously monitors IT environments to detect anomalies faster.
- Correlates data from multiple sources to reduce false positives.
- Provides predictive alerts, allowing teams to prevent issues proactively.
- Suggests and executes automated resolutions with minimal human oversight.
Core Features of AiOps Automation
AiOps platforms are built to handle the vast volume, velocity, and variety of data generated by modern IT ecosystems. By ingesting, analyzing, correlating, and acting on data in real time, AiOps brings both speed and accuracy to IT operations, ensuring fewer manual errors and much faster incident resolution.
Key Features That Enable AiOps Automation
- Automated Data Collection and Aggregation
- Pulls data from servers, applications, networks, security tools, and cloud platforms.
- Normalizes multi-source data into a unified format for easy correlation.
- Enables cross-domain insights, ensuring faster root cause analysis.
- Machine Learning-Powered Anomaly Detection
- Learns normal behavior patterns across systems, apps, and networks.
- Detects anomalies in performance, usage, and configuration.
- Flags potential issues before they cause service disruptions.
- Intelligent Alert Correlation
- Combines multiple alerts into unified incident records, reducing noise.
- Automatically classifies and prioritizes alerts based on severity.
- Reduces alert fatigue and helps teams focus on critical incidents.
- Automated Root Cause Analysis (RCA)
- Traces performance drops or failures across the full tech stack.
- Correlates logs, events, and metrics to find root causes in minutes.
- Provides historical context to predict potential recurrences.
- Automated Remediation and Self-Healing
- Triggers scripts to automatically fix known issues (restart processes, roll back changes, scale up resources).
- Supports policy-based automated responses, removing manual errors in remediation.
- Learns from successful past resolutions to improve future responses.
Benefits of AiOps: Saving Time and Eliminating Human Errors
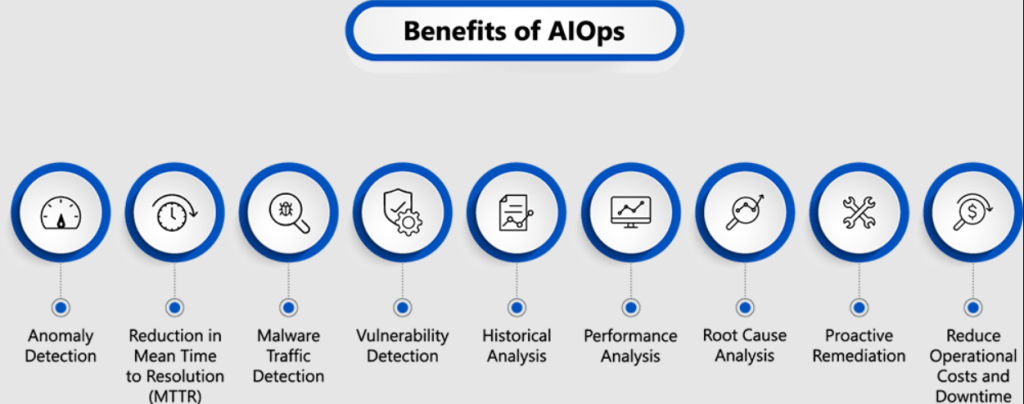
The power of AiOps Automation lies in its ability to speed up every phase of incident management while drastically reducing the risk of human error. Manual processes introduce errors at every stage—from initial detection to diagnosis and resolution. AiOps Automation solves this by intelligently automating the lifecycle of IT operations.
How AiOps Saves Time
- Real-Time Monitoring and Analysis
- Processes millions of data points in real-time across all layers.
- Detects issues within seconds, reducing time to detection (MTTD).
- Eliminates manual data aggregation and log analysis.
- Automated Diagnosis and RCA
- Correlates alerts, traces, and metrics to diagnose issues faster.
- Replaces manual root cause investigations with AI-driven insights.
- Reduces time spent on incident triage and correlation by up to 80%.
- Proactive and Predictive Alerts
- Predicts infrastructure failures based on historical patterns.
- Enables proactive responses, avoiding service-impacting incidents.
- Helps teams shift from reactive to predictive operations.
How AiOps Reduces Errors
- Removes Human Intervention from Routine Tasks
- Automates script execution, reducing misconfiguration risks.
- Standardizes remediation actions to ensure consistency.
- Eliminates Noise and False Alerts
- Uses AI to filter out irrelevant alerts, improving signal-to-noise ratio.
- Ensures only high-confidence alerts reach human operators.
- Prevents Recurring Issues
- Learns from previous incidents to recommend permanent fixes.
- Identifies systemic problems caused by poor configuration or workflows.
Real-World Use Cases of AiOps Automation
AiOps is already helping organizations across industries streamline operations, accelerate response times, and eliminate costly errors in critical IT environments. Here are some concrete examples of where AiOps delivers maximum value:
Practical Use Cases Where AiOps Saves Time and Reduces Errors
- Application Performance Management (APM)
- Tracks end-to-end app performance across cloud, on-prem, and hybrid environments.
- Detects code-level bottlenecks without manual performance testing.
- Suggests fixes directly to development teams.
- Cloud Cost Optimization
- Monitors multi-cloud deployments for cost inefficiencies.
- Automatically scales down unused resources, avoiding costly overprovisioning.
- Ensures that changes align with performance requirements.
- Infrastructure and Network Monitoring
- Monitors servers, containers, and networks in real time.
- Automatically flags and corrects configuration drift.
- Triggers auto-remediation workflows for common infrastructure faults.
- Security Operations (SecOps)
- Identifies anomalous behavior across networks, endpoints, and users.
- Correlates security alerts with operational incidents to prevent data loss.
- Automates threat containment actions, reducing reliance on manual security response.
- Compliance and Governance Monitoring
- Tracks infrastructure changes against compliance baselines.
- Automatically flags non-compliant configurations for correction.
- Reduces manual compliance reporting efforts.
Challenges in AiOps Implementation
While AiOps offers incredible promise, the road to full automation is not without obstacles. Organizations must address key technical, cultural, and operational challenges to fully reap the benefits.
Common Challenges When Adopting AiOps
- Data Integration Complexity
- Requires ingesting data from legacy systems, SaaS platforms, cloud providers, and custom apps.
- Data normalization is critical for accurate correlation.
- AI Model Training and Drift
- AiOps models must be trained on diverse operational data.
- Models can drift over time if not continually updated.
- Cultural Resistance
- IT teams may fear job displacement or lack trust in automated systems.
- Clear communication and upskilling programs are needed.
- Over-Automation Risks
- Blindly executing automated remediation without context can create cascading failures.
- AiOps must allow for manual intervention in complex scenarios.
- Compliance and Auditability
- Automated actions must be logged for audit purposes.
- AiOps tools must comply with industry regulations (GDPR, HIPAA, etc.).
The Future of AiOps: From Automation to Autonomous Operations
AiOps is just the beginning. As IT environments grow in complexity, AiOps will evolve from automation to fully autonomous IT operations (Self-Healing IT). Future AiOps platforms will:
What’s Next for AiOps?
- Predict and Resolve Incidents Before They Occur
- Digital twins and predictive modeling will allow AiOps to simulate outcomes.
- Deep Integration with DevOps Pipelines
- AiOps will become part of CI/CD processes, ensuring error-free releases.
- Generative AI for RCA and Documentation
- AI will automatically draft RCA reports, runbooks, and post-mortem summaries.
- Business-Aware AiOps
- AiOps will correlate technical incidents to business KPIs, prioritizing fixes with the highest business impact.