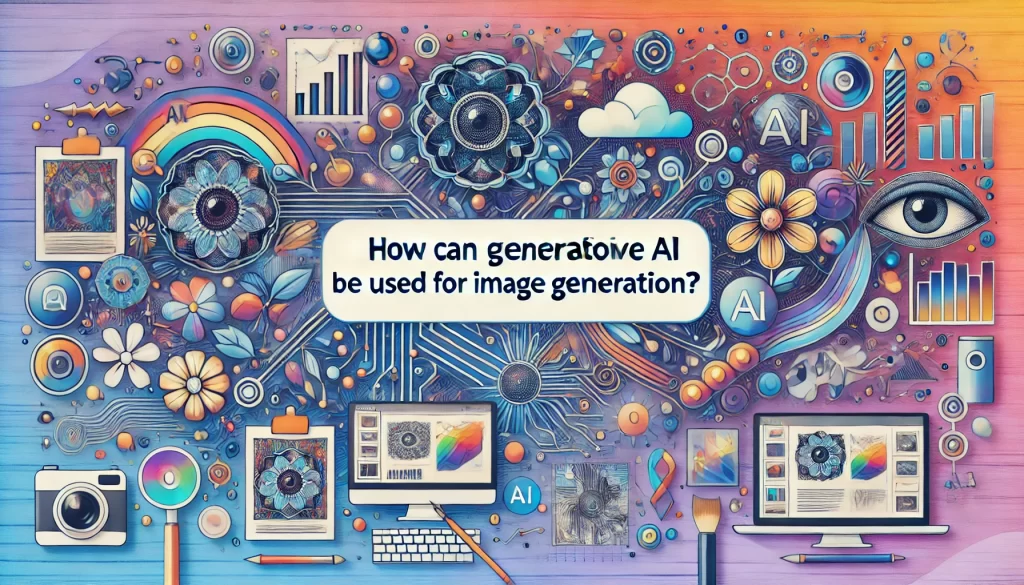
Generative AI has revolutionized image generation by enabling machines to create realistic, high-quality images from scratch or based on specific inputs. Using advanced model architectures, generative AI can produce everything from photorealistic images to imaginative art, often with minimal human guidance. This technology is proving valuable across industries, from entertainment and advertising to healthcare and design. Below, we explore how generative AI is used for image generation, covering key techniques, applications, and its transformative impact.
Key Techniques for Generative Image Creation
Generative AI uses several advanced techniques to create images. Each approach is designed to handle different aspects of image generation, from creating realistic textures to transforming one image style into another.
- Generative Adversarial Networks (GANs): GANs are one of the most popular and effective methods for generating high-quality images. GANs consist of two networks: a generator, which creates new images, and a discriminator, which evaluates the realism of these images. Through an adversarial feedback loop, GANs can produce incredibly realistic images.
- Variational Autoencoders (VAEs): VAEs are used to generate images by learning a compressed representation (latent space) of the data, allowing for creative image manipulation and synthesis. While VAEs donโt produce images as realistic as GANs, they are excellent for applications requiring smooth variations in image output, such as data augmentation and style interpolation.
- Transformers: Originally developed for text, transformer models have adapted for image generation in tasks like creating images from text prompts (e.g., DALL-E) or generating images based on specific context. Transformers use attention mechanisms to focus on specific parts of the data, making them effective for generating coherent and detailed images.
- Diffusion Models: Diffusion models generate images by simulating how patterns diffuse across pixels, gradually building a coherent image. These models have become popular in applications requiring high resolution and fine details.
Applications of Generative AI in Image Generation
Generative AI enables various innovative applications in image generation, offering both creative and practical solutions across industries.
a. Art and Design
- Generative AI can assist artists and designers by producing unique artworks, patterns, and illustrations based on prompts or inspirations.
- Applications include creating digital art, developing design concepts, generating patterns for fabrics, and producing visual content for social media.
- Example: Tools like DALL-E and Midjourney can turn text prompts into artistic images, allowing artists to experiment with new styles and visual ideas.
b. Advertising and Marketing
- Marketers use generative AI to create customized visuals for advertising campaigns, helping brands reach different audiences with tailored content.
- AI-generated images allow advertisers to quickly produce multiple variations of an ad or visual, A/B testing which design resonates best with customers.
- Example: An AI tool could generate different background environments for a product image, making it easy to adapt ads for different regions or demographics.
c. E-commerce and Virtual Try-Ons
- In e-commerce, generative AI creates realistic product images or virtual try-on experiences, helping customers visualize how products like clothing or furniture will look in real life.
- Retailers can also use AI-generated images for product mock-ups, allowing potential buyers to customize designs.
- Example: Virtual try-on apps use generative AI to allow users to โtry onโ clothing, accessories, or makeup, enhancing the online shopping experience.
d. Entertainment and Gaming
- Game developers use generative AI to create dynamic, procedurally generated environments, characters, and assets for immersive gameplay.
- AI can also generate background scenes, textures, and even entire landscapes, saving time and offering endless variation.
- Example: A game might use AI to automatically generate new, unique landscapes every time a player enters a new area, enhancing replayability and immersion.
e. Healthcare and Medical Imaging
- In healthcare, generative AI is used to create synthetic medical images, such as MRI or CT scans, for research, training, or data augmentation. Synthetic images help medical professionals and researchers study rare conditions without needing a large dataset of real patient data.
- Example: Generative models can produce a range of CT scans that simulate a specific condition, aiding medical researchers in developing diagnostic tools and training medical students.
f. Fashion and Design Prototyping
- Fashion designers leverage generative AI to quickly prototype clothing designs, patterns, and textiles. AI can generate variations of an outfit based on certain style constraints, saving time and resources during the design process.
- Example: A fashion designer might input specific style parameters into an AI model to generate multiple dress designs, iterating quickly to find the best concept.
g. Augmented Reality (AR) and Virtual Reality (VR)
- In AR and VR, generative AI helps create realistic or stylized 3D environments, objects, and textures, providing more immersive virtual experiences.
- These AI-generated visuals can be used in training simulations, virtual tourism, and virtual social spaces, allowing for richer and more interactive virtual environments.
- Example: A virtual tourism app might use generative AI to create lifelike historical scenes, allowing users to experience ancient landmarks in VR.
The Process of Training Generative AI for Image Generation
Training generative models for image generation involves several key steps to ensure high-quality output.
- Data Collection: The model requires a large dataset of images representative of the content it needs to generate. For instance, a model designed to create portraits would need a dataset of high-quality human faces.
- Preprocessing: The images are standardized, resized, and formatted to be suitable for the model. This might involve data augmentation, such as rotating or flipping images, to increase dataset diversity.
- Model Training: The model (e.g., GAN, VAE) learns to generate images through iterative training, adjusting its parameters based on loss functions and feedback loops.
- Fine-Tuning: Hyperparameters like learning rate, batch size, and layer depth are adjusted for optimal output quality. In GANs, the generator and discriminator are fine-tuned to balance each other effectively.
- Evaluation: Metrics like Frechet Inception Distance (FID) assess the quality and realism of generated images. Human evaluation is also common, especially for creative applications, to ensure the images meet quality standards.
Benefits of Generative AI in Image Generation
Generative AI offers several advantages in image generation:
- Efficiency: Reduces the time and effort needed to create high-quality visuals, enabling creators to produce a wide range of images quickly.
- Cost Savings: Minimizes the need for extensive photo shoots or manual design, particularly in advertising, fashion, and product prototyping.
- Customization: Allows users to generate images tailored to specific preferences or styles, enhancing personalization.
- Innovation: Enables artists and designers to experiment with new concepts, styles, and compositions, pushing the boundaries of creativity.
Challenges and Ethical Considerations
While generative AI is powerful, it comes with challenges:
- Bias in Training Data: If the training data lacks diversity, generated images may reflect biases, limiting their applicability or inclusiveness.
- Intellectual Property Concerns: Generative models trained on copyrighted images raise ethical questions about ownership and originality, especially in commercial use.
- Deepfake and Misinformation Risks: AI-generated images can be used to create deepfakes or misleading content, posing risks for privacy, security, and public trust.