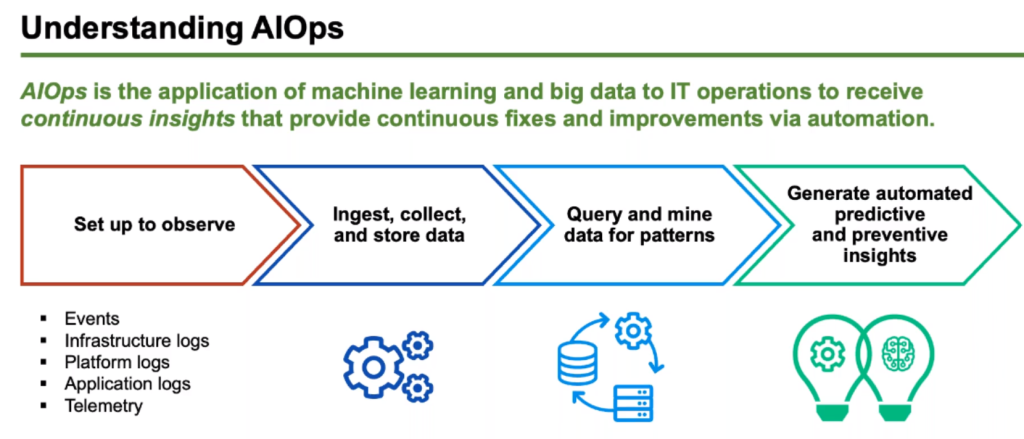
There are a number of different ways to measure the success of AIOps. Some of the most common metrics include:
- Mean time to detect (MTTD): This metric measures the time it takes to detect an issue. A lower MTTD indicates that AIOps is effective at detecting problems early.
- Mean time to resolve (MTTR): This metric measures the time it takes to resolve an issue. A lower MTTR indicates that AIOps is effective at resolving problems quickly.
- Number of incidents: This metric measures the number of incidents that occur. A lower number of incidents indicates that AIOps is effective at preventing problems.
- Cost of incidents: This metric measures the cost of incidents. A lower cost of incidents indicates that AIOps is effective at reducing the financial impact of problems.
- Customer satisfaction: This metric measures the satisfaction of customers with the IT services. A higher customer satisfaction indicates that AIOps is effective at improving the quality of IT services.
Measuring the success of AIOps (Artificial Intelligence for IT Operations) implementation involves assessing how effectively the solution is achieving its intended goals and delivering value to your organization. Here are some key metrics and approaches to measure the success of AIOps:
- Incident Response Time:
- Measure the time it takes to detect and resolve incidents before and after AIOps implementation. A reduction in incident response time indicates improved operational efficiency.
- Downtime Reduction:
- Track the frequency and duration of system downtime or outages. A successful AIOps implementation should lead to a decrease in unplanned downtime.
- Mean Time to Repair (MTTR):
- MTTR measures the average time taken to resolve incidents. A shorter MTTR indicates improved incident management efficiency.
- Anomaly Detection Accuracy:
- Evaluate the accuracy of AIOps in detecting anomalies and predicting incidents. Compare true positives and false positives to assess the system’s performance.
- Predictive Accuracy:
- Measure the accuracy of predictions made by AIOps against actual outcomes. Compare predicted incidents with incidents that actually occurred.
- Resource Utilization Optimization:
- Assess whether AIOps recommendations for resource allocation and optimization are leading to better utilization and cost savings.
- Cost Savings:
- Calculate the cost savings achieved through improved resource utilization, reduced downtime, and optimized cloud spending (if applicable).
- Automated Remediation Rate:
- Measure the percentage of incidents that are automatically resolved by AIOps without human intervention.
- Incident Escalation Rate:
- Track the percentage of incidents that require escalation to higher-level support or management. A decrease indicates better incident resolution at lower levels.
- Business Impact Analysis:
- Evaluate how well AIOps can assess the impact of incidents on business processes and user experience.
- User Satisfaction and Experience:
- Gather feedback from IT teams and end users to gauge their satisfaction with the improved IT services and reduced disruptions.
- Operational Efficiency:
- Analyze IT operations team productivity and efficiency before and after AIOps implementation. Are teams able to focus more on strategic tasks?
- Model Performance Metrics:
- For predictive and anomaly detection models, track metrics such as precision, recall, F1-score, and area under the ROC curve to assess their effectiveness.
- Feedback and Adaptation:
- Monitor how often AIOps algorithms and models are updated based on user feedback and changing IT environments. Frequent updates can indicate a responsive system.
- Alignment with Business Goals:
- Ensure that AIOps outcomes align with the organization’s business objectives, such as improved customer experience, faster time-to-market, or reduced operational costs.
- Continuous Improvement Initiatives:
- Track the number and impact of improvements made to the AIOps system over time, demonstrating a commitment to refining and enhancing the solution.
1. Mean time to detect (MTTD)
MTTD measures the time taken to identify the issue. AIOps detects patterns and groups events, sifts signals out of noise, and reduces event streams up to 95% to identify the critical alerts related to IT infrastructure performance. Hence, AIOps leads to faster anomaly detection, reduced downtime, and enhanced productivity.
2. Mean time to acknowledge (MTTA)
When an issue is detected, IT teams must acknowledge the problem and identify who will resolve it. AIOps uses machine learning algorithms to automatically decide who will address the issue and ensure the right people are up and working on it.
3. Mean time to resolve/repair (MTTR)
Time is money, and when an essential process or app is down, getting it up and running on time is crucial. MTTR measures the average time required to repair faulty equipment. Simply put, it is the time that lapses between the start of the incident and when the system returns to full functionality.By diagnosing the root cause of the issue and escalating the problem to the right team of IT professionals, AIOps reduces the MTTR. Using machine learning, the systems can quickly identify whether an issue has occurred in the past and recommend/automate actions to resolve it.
4. Ticket-to-incident ratio
Often, tens or hundreds of tickets are raised for the same issue, especially if the anomalous event has a cross-stack impact. In such a situation, tickets seldom map to incidents in a 1:1 ratio. While different teams are investigating the incident from varied perspectives, organizations must be mindful of the time it takes to realize it is the same problem.AIOps correlates and groups the data generated from multiple IT environments to reduce the number of tickets, logs, and events and diagnose problems swiftly, thus improving the service desk’s efficiency and freeing up staff to focus on other value-adding tasks.
5. Service availability
Service availability refers to the percentage of uptime over a specific period of time. Simply stated, it is the outage minutes per period of time.Machine learning algorithms analyze past data to predict and resolve potential network downtime and prevent business-critical outages. Moreover, AIOps can address the less-urgent alerts pertaining to more urgent issues before they cause severe harm.
6. Mean time between failures (MTBF)
The mean time between failures or MTBF means the average time between system breakdowns. MTBF is calculated by dividing the number of operational hours by the number of failures. For example, an asset operates 1,000 hours a year, and in the previous year, it broke down eight times. Therefore, MTBF for that asset is 125 hours.Needless to say, AIOps helps improve MTBF by rectifying current issues and predicting potential future outages.
7. Automated vs. manual resolution
Machine learning algorithms can identify patterns, learn from past remediation measures taken, e.g., previous scripts executed, and automatically remedy the problem, thus reducing the need for manual intervention.
8. User reporting vs. automatic detection
IT teams must detect and resolve issues before the end-user becomes aware of them and reports them to the company.AIOps leverages dynamic thresholds for automated alert generation and escalation to remedy problems before the end-user is affected.
9. Common business KPIs
AIOps is inevitably a vital business asset that helps improve typical business KPIs. By ensuring network stability and minimizing downtime, AIOps streamlines revenue cycle and operations. Additionally, it enhances the service quality of business apps that customers use, improving customer experience and building customer trust and loyalty in the process. Moreover, AIOps diagnoses anomalies, sends specific alerts to the IT team, and assists them in predicting future outages. This helps your IT staff be more productive and focus on tasks that fuel business growth.
Here are some additional tips for measuring the success of AIOps:
- Set clear goals and objectives. Before you start measuring the success of AIOps, it’s important to set clear goals and objectives. This will help you to determine which metrics are most important to track.Choose the right metrics. There are a number of different metrics that you can use to measure the success of AIOps. Choose the metrics that are most relevant to your goals and objectives.Collect data consistently. In order to get accurate results, it’s important to collect data consistently. This means collecting data at regular intervals and from the same sources.Analyze the data regularly. Once you have collected data, you need to analyze it regularly to track the progress of your AIOps initiatives.Make adjustments as needed. As you analyze the data, you may need to make adjustments to your AIOps strategies. This is normal, as AIOps is a continuous improvement process.